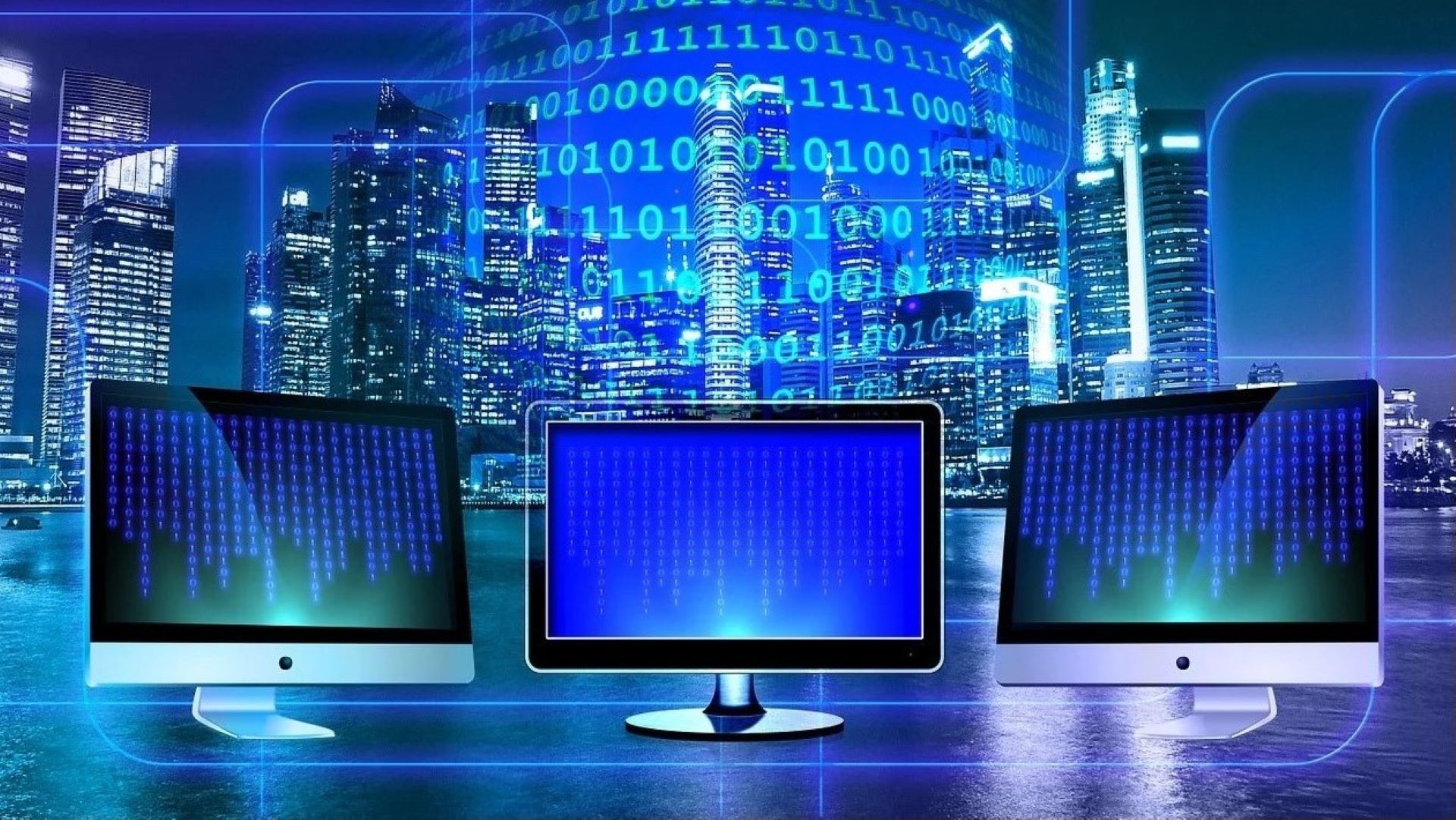
An analysis from Goldman Sachs Economics Research recently compared the ongoing AI boom to the advent of electricity and personal computers, stating that innovations in these areas boosted U.S. GDP by as much as 2% as the productivity gains made their way through the broader economy. Today, Goldman Sachs sees AI boosting global labor productivity by 1% annually in the decades following its widespread usage.
However, a new survey from Capital One finds a substantial number of companies with a considerable amount of technical and organizational work ahead of them if those potential productivity gains are to be realized.
For instance, the Capital One survey on AI readiness revealed a significant disconnect between business leaders’ perceptions and the operational realities faced by technical practitioners. The study, which surveyed 4,000 business leaders and technical practitioners across various industries, also highlights several key challenges in implementing AI at scale.
Notably, the survey exposes a stark contrast between leadership confidence and technical on-the-ground realities. While 87% of business leaders believe their organizations have modern data ecosystems capable of building and deploying AI solutions at scale, 70% of technical practitioners report spending up to four hours daily fixing data issues, conducting quality checks, or correcting errors. “It appears that people haven’t made the connections between how important data management and having a data culture is to success,” said Terren Peterson, VP of data engineering at Capital One, in an interview with TechStrong.ai. “Spending up to four hours a day doing data clean-up is a real eye-opener, and it points to how much, or little, time they are investing into data hygiene,” he said.
Data culture also emerges as a crucial factor for AI success, yet the survey also reveals a significant shortfall in this area. Only 35% of respondents report having a strong data culture, with over 20% indicating a lack of strong data culture or inconsistent leadership support, talent development, and education around data. This disconnect between recognizing the importance of data culture and fostering it within organizations is a major hurdle to AI readiness. “Companies are going to have to come to terms with and that, with the focus on AI, they have another reason to remind them that good data management needs to be prioritized,” Peterson said.
The survey also uncovers a concerning lack of alignment on AI strategy. While 78% of tech practitioners and 82% of leaders agree on the importance of an AI strategy, only 53% of tech practitioners and 55% of leaders are fully familiar with their organization’s AI strategy. This misalignment could lead to ineffective implementation and hinder the success of AI initiatives.
“I think this result could be a classic perspective of strategy versus execution,” added Peterson. “Business leaders are thinking of the strategy and how important it is, and they know that they’ve socialized it, they’ve made technology investments in this area, and they believe that the benefits are going to come. But the practitioners, the ones that are on the front lines of that, they’re executing and they’ve been through other generations of technology waves, and they’re seeing that there’s a lot of heavy lifting still to be done.”
Technical capabilities present another significant challenge. Only 36% of tech practitioners and 47% of business leaders strongly agree that their organizations have the necessary skills and expertise for complex AI projects. Furthermore, just 51% of both groups are confident in the scalability of their AI solutions, and only 33% of tech practitioners and 41% of business leaders report successfully scaling AI-driven solutions across their enterprises.
These findings suggest that while there is strong intent to embrace AI, many organizations are falling short in execution and implementation. Companies that address these challenges, including improving data management, fostering a robust data culture, and acquiring the right technical skills, will be better positioned to leverage AI’s full potential and achieve meaningful business outcomes in the long term.
This also aligns with Goldman Sachs’ findings that, for large scale AI transformation to occur, businesses will need to make significant upfront investment in physical, digital, and human capital to acquire and implement new technologies and reshape business processes. Goldman Sachs estimates that those investments, which could amount to around $200 billion globally by 2025, will probably happen before adoption and efficiency gains start driving major gains in productivity.
As AI continues to evolve, and early-adopters make gains in AI-driven productivity, organizations that fail to bridge this readiness gap will find themselves at a significant competitive disadvantage.