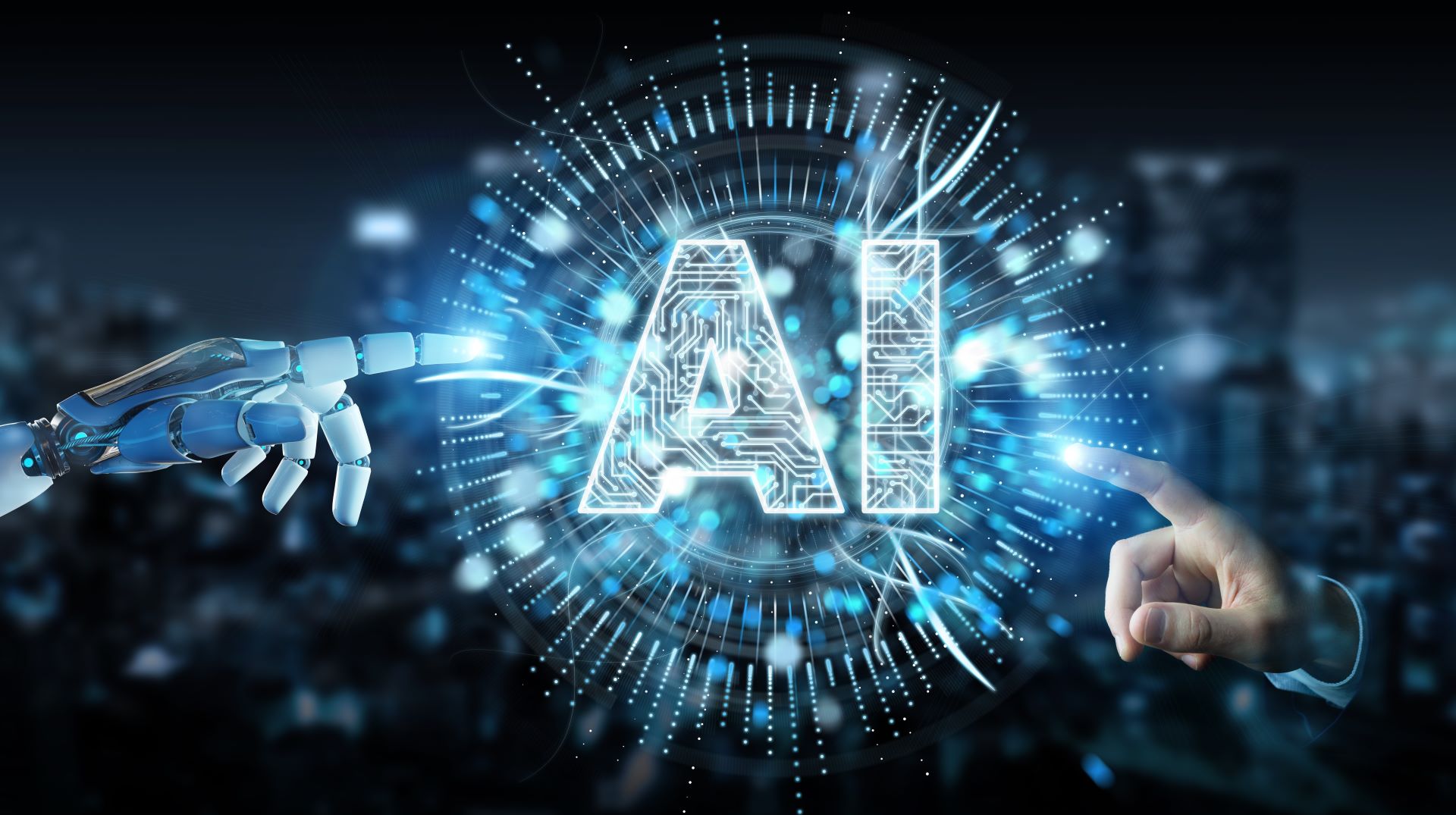
Generative AI adoption has increased like wildfire in the past year as more organizations explore its capability to boost business productivity. However, given the rapid evolution of GenAI technology, business leaders face a critical problem: How to select the right type of solutions.
Currently, business leaders have two options when it comes to integrating GenAI capabilities within their workforce: Commercial AI services and open source large language models (LLMs). Each brings its unique advantages and challenges. Options like the state-of-the-art LLMs, ChatGPT, or the more enterprise-centric Azure OpenAI Service from Microsoft provide integration-ready solutions, but entail single-provider risks, as well as concerns about data leakage and intellectual property protection.
On the other hand, open source LLMs offer customization possibilities, aligning closely with specific business needs and potentially yielding more precise results. However, integrating these open source solutions requires higher operational costs and specific skill requirements. For business leaders, the dilemma boils down to prioritizing time-to-market or data protection.
The latest survey from McKinsey revealed that 40% of C-suite executives think their organizations will increase investments in GenAI in 2024. So, with AI at the top of the agenda, how can businesses take a more balanced approach when selecting the optimal solution for their workforce?
Evaluating Open Source vs Commercial GenAI Options
Commercial GenAI services provide a lower entry barrier for businesses. They are ready-made for enterprises, where users can simply log in and start using them without any significant time or resources required for deployment. Some commercial GenAI services also provide strong security controls and compliance with data regulations though many lack verifiable data governance and protection assurances regarding confidential enterprise information. Information entered into these commercial providers for example can become part of their training set. Proprietary, sensitive or confidential information entered as prompts may be used in outputs for other users. Additionally, the lack of content filtering for specific enterprise policies and the potential for generating inaccurate responses are significant drawbacks. One study into ChatGPT found that it was able to answer 16 out of 21 questions with the expected level of accuracy, with the main issue being a tendency towards more conservative answers than expected from a human.
Conversely, open source LLMs such as, Mistral, BLOOM and GPT-J offer a different value proposition. They provide greater flexibility for customization, allowing businesses to fine-tune AI models to their specific needs, resulting in more accurate and relevant outputs. With open source LLMs, businesses can build their own LLM chatbot for specific tasks, whether it’s content generation, programming, data analytics or any administrative tasks. They also provide the most flexibility for enabling security and protections allowing firms to implement security controls based on data classification, data protection and role-based access management.
Open source options, however, often require significant operational investment, both in terms of infrastructure and the specialized talent needed to customize or manage these systems effectively. Organizations will need to be particularly careful when inputting data to train the system or risk a badly trained AI that produces skewed results. This can be a lengthy and manually intensive process.
The decision between these two paths is influenced by several factors, including data privacy implications, cost considerations and the desired level of control over the AI system. Understanding these trade-offs is crucial for businesses to make an informed decision that aligns with strategic objectives such as increased productivity or launching new services as well as their risk appetite.
Understanding the Security Risks
For commercial AI services, the main security concerns revolve around their handling of sensitive data. While these services generally provide robust security infrastructure, they don’t inherently protect data and confidential information in conversations. Enterprises should put controls in place to avoid inadvertently exposing enterprise IP directly in commercial LLMs.
Open source AI models present a different security challenge. Lacking the comprehensive security infrastructure of commercial platforms, these models require businesses to establish and maintain robust security protocols independently. This includes developing defenses against prompt injection attacks and setting specific access policies and authentication protocols.
The legal and regulatory considerations are also critical in this choice. For commercial AI services, businesses must consider how these services comply with international data laws and regulations. For instance, Microsoft’s compliance with EU data residency laws, which require data on EU citizens to be stored within the EU, can be a significant factor for businesses operating in or dealing with European customers. Additionally, the use of commercial AI services involves the navigation of contractual nuances, especially around liability for data breaches or misuse of AI-generated content. LLMs often fall short when it comes to privacy and data leakage, which can be a serious problem for firms inputting proprietary and confidential information. There have also been legal issues over data used to train LLM models, such as a coalition of authors suing OpenAI for allegedly using their work without permission.
In contrast, open source AI solutions place the onus of regulatory compliance directly on the businesses that deploy them. This includes ensuring adherence to copyright laws, as the responsibility for any copyright infringement arising from AI outputs falls on the users. Furthermore, businesses using open-source AI must stay informed on global regulatory changes, such as new AI guidelines or restrictions, which can vary significantly across regions.
Given the dynamic nature of AI regulation, businesses must be proactive in understanding and adhering to the legal requirements relevant to their chosen AI solution. This involves regular consultations with legal experts, especially when operating across multiple jurisdictions, to ensure ongoing compliance and mitigate legal risks.
Guidance for a Balanced Approach
Given the complexities surrounding both commercial and open source GenAI options, business leaders must make strategic choices that align with their operational needs, security posture and compliance requirements. For businesses seeking maximum control over their AI applications, hosting open source LLMs offers significant flexibility. However, this approach requires a robust internal infrastructure for operational management and security. Businesses must be prepared to invest in the necessary talent and security measures to mitigate risks effectively.
When prioritizing security and compliance, commercial options like Microsoft Azure OpenAI Service are advisable. At the same time, businesses should supplement these services with additional internal controls to offset potential limitations, such as content filtering and managing AI-generated inaccuracies.
Regardless of which option business leaders go for, implementing third-party or homegrown content filtering systems is crucial. These systems should screen prompts and outputs against enterprise policies and legal compliance requirements, including copyright considerations. Security teams should also leverage layered security measures based on data classification, protection protocols and role-based access management. These measures should be tailored to address the specific security needs of the chosen AI solution.
Most importantly, business leaders must continuously monitor and adapt to the evolving legal and regulatory landscape of AI technologies. This involves regular consultations with legal experts to ensure ongoing compliance and to safeguard against potential legal challenges.
Ultimately, the choice of which AI tools to use and how to deploy them depends on each organization’s unique needs and capabilities, underscoring the importance of a strategic approach to AI integration in the modern business environment.