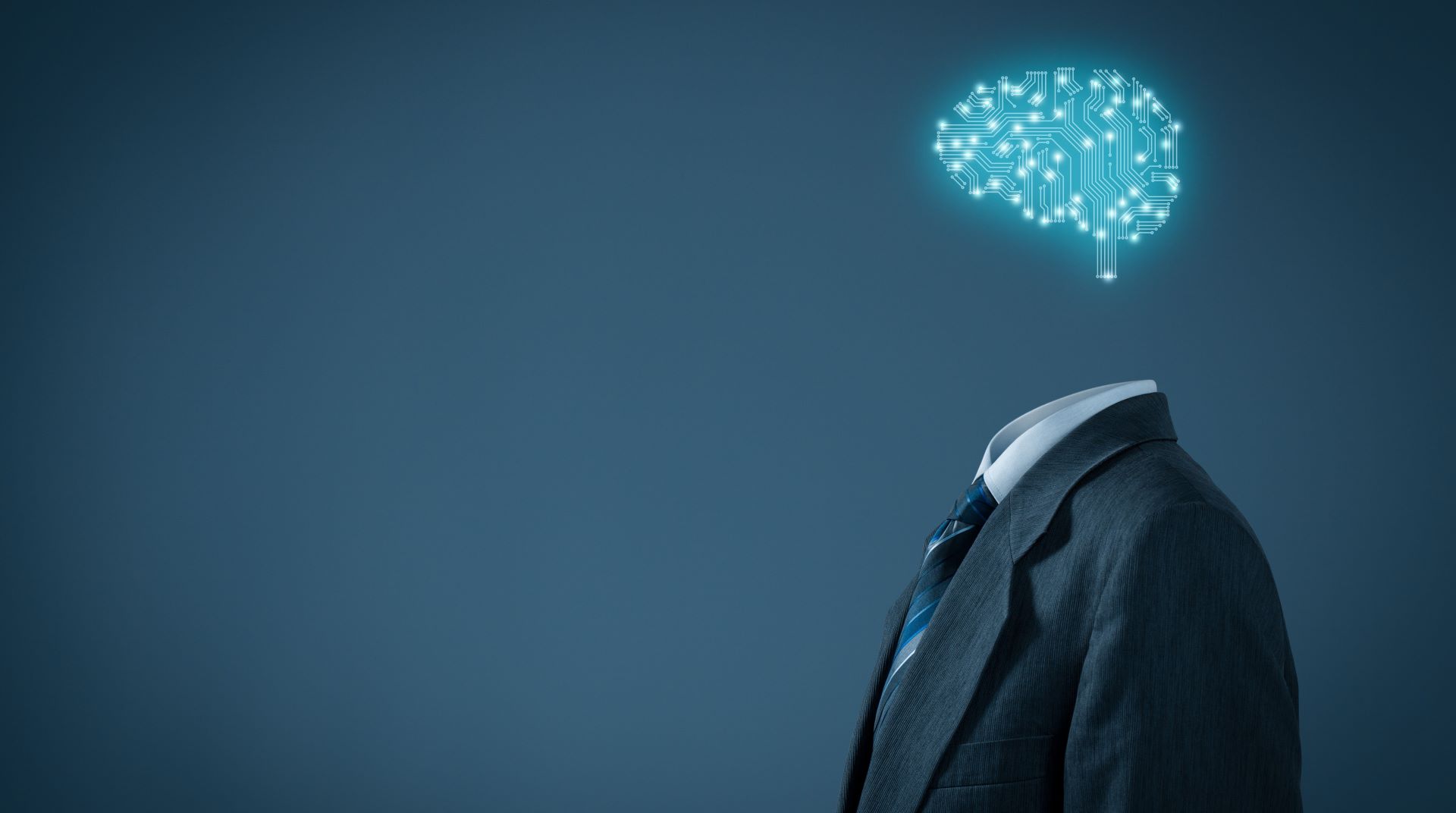
Poet Robert Browning wrote that a man’s reach should exceed his grasp. But maybe it’s not always such a good idea for an enterprise to exceed its grasp when striving to achieve new things — at least when it comes to implementing something still mystery-filled, such as generative AI.
While tech giants like Microsoft, Google, Salesforce and others have rushed to deploy generative AI products, enterprises are also eying ways to deploy their own AI. But moving too quickly is fraught with pitfalls and risk. The results have fallen short of stellar at times. Various public-facing generative AIs have “lied” about the background of individuals, threatened users and provided errored responses with great confidence.
CxOs often make the mistake of underestimating the complexity of generative AI and its potential impact on their business. They may also overlook the need for investment in infrastructure, data management and talent acquisition. Additionally, they may not fully understand the ethical implications of generative AI and fail to establish guidelines for its use.
“Rushing into the hype and excitement of new technologies concerns me,” says CF Su, VP of machine learning at Hyperscience. “Platforms like OpenAI’s ChatGPT and Google’s Bard were pushed to market much faster than they should have been—just look at the mistake Bard made during its initial demo that cost its parent company over 9% in stock value overnight. Companies must prioritize accuracy over speed as they push forward with implementation plans.
“The worst mistake enterprises can make now is to have too much trust in generative AI,” Marcin Stoll, chief product officer at Tidio, advises. “It’s still new, and it’s still not perfect. It’s vital to monitor everything it produces and put the trust into humans that operate the AI, not the opposite,” says Stoll.
We contacted industry experts to learn the top mistakes enterprises will likely make when deploying generative AI independently. Below are the top potential pitfalls they identified.
The Data Integrity Pitfall
It doesn’t matter how good the underlying AI models are (they do need improvement to stop hallucinations); if the underlying data is problematic, the results will be difficult. We’ve recently covered how enterprises don’t always trust their data and that enterprises must avoid the rush into a generative AI deployment with poor data or data the organization can’t trust.
“Any ML or AI model is very dependent on the quality of the underlying data and how well it represents the real world. If we force an ML or AI algorithm or service to learn from sub-optimal data, the quality of the results could be insignificant,” says Jayaprakash Nair, head of analytics at Altimetrik.
Joe Atkinson, chief products and technology officer at PwC, agrees. “The quality of the data used to train generative AI models is critical to their success. The model will produce flawed results if the data is incomplete, biased or inaccurate. Enterprises should ensure that their data is quality and up-to-date to avoid this problem,” says Atkinson.
The Technical Readiness Pitfall
In addition to the quality of enterprise data, enterprises must ensure they have the technical readiness regarding infrastructure and talent to handle and manage their systems effectively. An infrastructure that handles data-intensive AI workloads demands enough storage, extremely low latency I/O, robust networking capabilities and big data and analytics capabilities.
“Not every organization will have the on-hand resources to fully realize the business value of ChatGPT,” says Bart Schouer, chief evangelist of IoT and analytics at Software AG. “This is especially true if they don’t have sufficient engineers, time and resources to develop the right applications and integrations on their own to maximize value and ensure its effectiveness,” says Schouer.
Adam Segall, an analyst at GP Bullhound, says that a lack of implementation strategy can lead to wasted resources, misalignment and unintended outcomes. “While the promise and capabilities are certainly great, unrealistic expectations can lead to disappointment and frustration – for now,” adds Segall.
The Total Cost Pitfall
Brandon Jung, VP of ecosystem at Tabnine, says stand-alone AI engineering companies “require available infrastructure capital to train AI at a reasonable cost and maintain viability.”
“Generative AI models must be integrated with existing enterprise systems to be effective. This can be a complex and time-consuming process that requires careful planning and coordination,” adds Atkinson.
Additionally, adds Atkinson, “Generative AI is a complex field that is changing quickly. Organizations should help ensure that nearly all employees develop a basic knowledge of generative AI. Educating your employees—not only on the technology but also on responsible use—will be critical to developing AI models that are successful and effective,” says Atkinson.
The Security and Governance Pitfall
If enterprises aren’t careful, security breaches involving the data that feeds into the model and enterprise queries and results could be breached. “One of the biggest questions I get is about the security implications of generative AI in the workplace. Before March 1, if you supplied ChatGPT corporate secrets, those could very well show up in other queries, like your competitors or a nation-state,” says Matt Chiodi, chief trust officer at Cerby.
Such leaks have already occurred. Earlier this month, employees of technical giant Samsung reportedly entered security data into ChatGPT. Further, lawyers warn that companies may lose trade secret protections by sharing data with ChatGPT, which may mean such data is no longer technically secret.
“People should think about what controls are or are not in place to help protect them from manipulation, coercion or harm. Once you realize how much is already happening, it demonstrates the obvious and necessary importance of governance over these AI systems,” concludes Andrew Clark, CTO and co-founder of Monitaur.
The best way to avoid these pitfalls and move forward successfully with generative AI deployments is to make sure the enterprise is first ready for generative AI deployment. Typically, the first hurdle will be ensuring the quality and integrity of the data used to feed and care for the AI. The infrastructure, tools and appropriate talent level must be in place. Finally, legal and security concerns must be addressed before any widescale deployment with regulated or sensitive information.
Finally, CxOs should be prepared to iterate and refine the model continually to ensure its accuracy and relevance over time. “Successful generative AI programs balance value with responsibility. High risk may sometimes mean high reward, but this mantra does not apply to AI solutions. While immense value can be created by leveraging these tools, employee, customer and public trust should remain a guiding principle,” Don Schuerman, CTO at Pega, contends.
“There is a lot of room for improvement, so it’s crucial to allow for this improvement and the mistakes that AI will inevitably make. The most important thing is to ensure that those mistakes will not cost your business too much,” concluded Tidio’s TK.
This is great advice. After all, enterprises shouldn’t gamble their fate and design AIs whose reach exceeds their grasp.