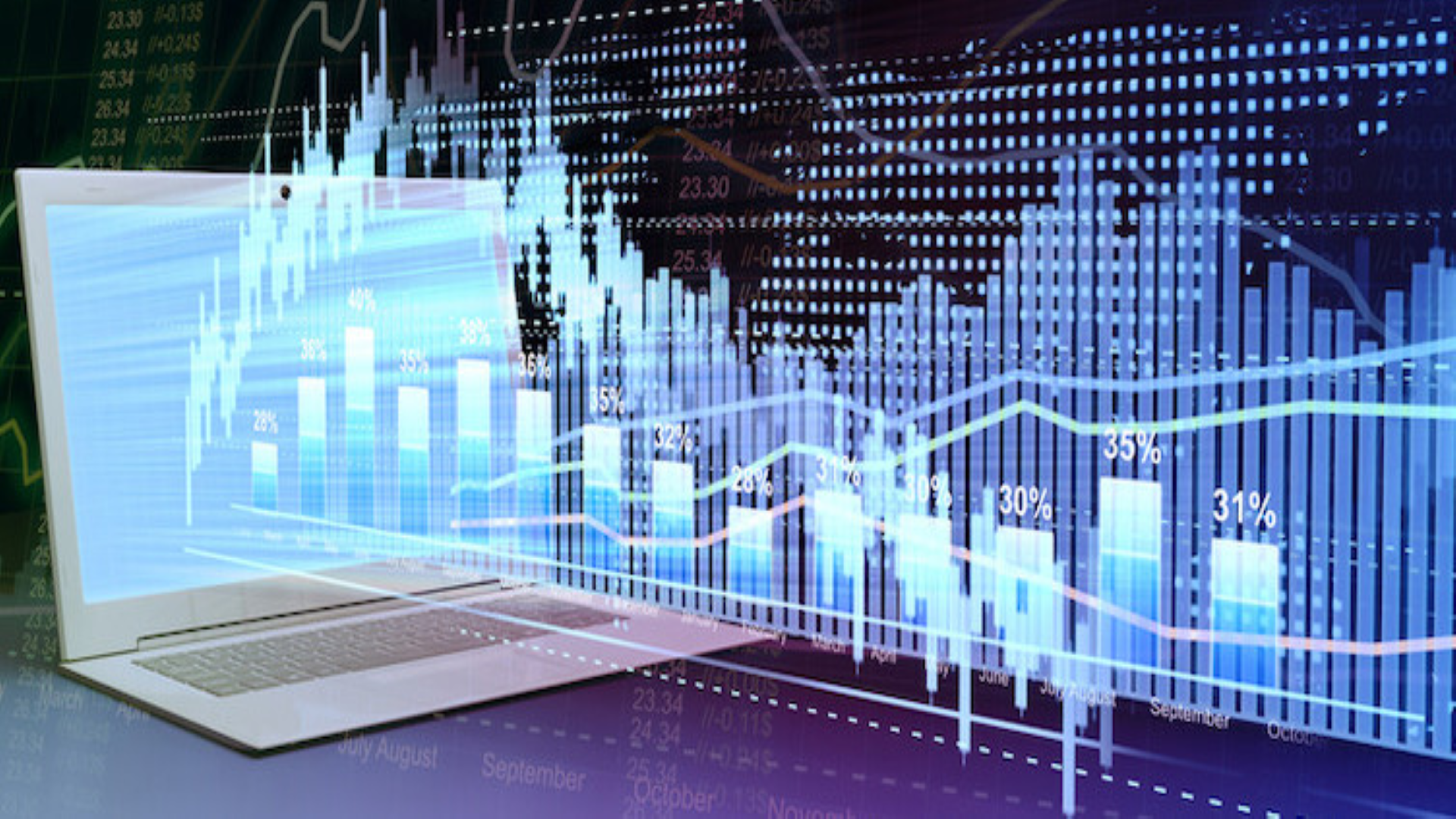
Considering the vast amount of business data and information permeating the web nowadays, big data analytics solutions have become indispensable for businesses trying to gain a competitive edge.
Nowadays, the world’s stored data has amassed to being almost entirely digital, from quarterly financial projections and customer purchasing history to sales and marketing reports and audio-visual files. A big data analytics solution contains a suite of powerful technologies and tools that enable organizations to extract valuable insights from huge amounts of granular data.
This can then be used to drive innovation in their sales, marketing, lead generation and growth strategies with informed decision-making at the epicenter. Big data examples vary from application to application, but the salient purpose of them is to help users make critical business decisions with improved confidence.
This article explores the influence that big data and analytics solutions have on organizations today, their common applications and how they can inform new business strategies.
Understanding Big Data Predictive Analytics Solutions
What is big data analytics?
Big data analytics software solutions analyze data sets to uncover hidden insights, unknown correlations, consumer preferences, market trends and helpful patterns. Open-source advanced analytics solutions like those involved in analyzing large amounts of data encompass a wide range of techniques and technologies within them to help organizations make data-driven decisions.
Difference between big data and traditional data analysis
While traditional data analytics typically involves smaller, structured data sets, big data analytics solution providers deploy enterprise-grade solutions designed to process data that is categorized as both structured and unstructured. The key differences lie in the scale, diversity and complexity of the data being analyzed, with organizations striving for agility and growth often pursuing big data predictive analytics solutions that can scale accordingly.
The Role of a Big Data Scientist or Analyst
Big data analysts and those with similar titles would be responsible for collecting, processing and assessing large amounts of data through a cloud-based analytics platform.
These individuals would adopt principles of data science and big data analytics making data-driven decisions, using advanced machine learning (ML), artificial intelligence (AI) and predictive modeling to extract meaningful insights that can inform strategies and decision-making processes.
Types of Big Data
Structured data
Structured data categorizes that which is highly organized and easily searchable. This type of data is usually stored in relational databases, spreadsheets, documents or other shared systems. Such examples include customer information, inventory data, sales figures, and transaction records, among others.
Unstructured data
This type of data lacks a predefined data model, hence its name, and thus is more challenging to analyze and process. Unstructured data types include text documents, social media posts, images, videos, customer reviews and emails. However, thanks to AI/ML, it’s possible to gain valuable insights from this vast category of data.
Semi-structured data
Semi-structured data falls between structured and unstructured data, containing tags and labels (metadata) but not conforming to a rigid structure. Examples include XML files, JSON data, and archive or log files.
Metadata
Metadata describes other data, often providing characteristics, attributes and properties of a dataset. This could include the duration, encoding and resolution of video file formats, or timestamps and location data of a social media post.
Other types of big data include time-series data to analyze patterns, trends and changes like stock market prices, IoT sensor readings, website traffic and weather data. Geospatial data includes coordinates, addresses, maps and satellite data, often used in GPS navigation systems and environmental monitoring.
Aspects of Big Data Analytics Solutions
- Data storage and management: Big data analytics solutions rely heavily on efficient storage and lifecycle management. Solutions often include data warehouses or centers to store and organize vast quantities and categories of data.
- Data processing and analysis tools: Big data solutions utilize powerful processing tools like Apache Hadoop, Apache Spark, and various SQL databases.
- Reporting: Effective data visualization tools are essential for presenting complex insights in an easily understandable format.
Benefits of Big Data Analytics Tools
The benefits of big data analytics are well-documented, and organizations of varying industries and sizes can unlock potential in numerous aspects of their operations. These solutions empower organizations to:
- Make informed and accurate decisions
- Identify inefficiencies or bottlenecks in their processes
- Optimize their costs
- Gain deeper insights into consumer behavior
- Create more targeted marketing campaigns
- Facilitate aggregation of analytics in e-commerce platforms
- Improve customer experiences across touchpoints
- Forecast trends with confidence
- Identify and address challenges and risks
Big Data Analytics Problems and Solutions
With the right approach and methodology, organizations will be able to take onboard insights derived from big data to enhance their operational agility and make critical business decisions with greater confidence. However, big data problems can arise from time to time, particularly if large-scale solutions have not been deployed in an incumbent environment before.
Many organizations encounter challenges in ensuring data quality and a seamless integration across diverse sources. Data governance policies and frameworks make this process much easier across the board. Others find that as data volumes grow, maintaining adequate system performance and uptime becomes vital. Cloud-based data analytics solutions, unified platforms, and distributed frameworks all offer scalability to address thisand other challenges.
Protecting sensitive data is vital which is why robust security measures and compliance with relevant regulations are crucial when deploying big data solutions. Among the most influential regulations are the General Data Protection Regulation (GDPR) and the California Consumer Privacy Act (CCPA), enacted in 2018 and 2020 respectively. Organizations are encouraged to stay updated and proactive in implementing relevant tools, policies and solutions that comply with these relevant regulations as far as data collection, maintenance and distribution are concerned. Failing to do so results in hefty penalties and fines, not to mention damage to an organization’s reputation and consumer trust.
Industry-Specific Applications
Health care:
In the health care space, big data analytics can improve resource allocation, enhance research capabilities, and improve patient outcomes. Robust security measures and protocols are vital to ensure patient data remains secure and encrypted at rest and in transit.
Finance:
Financial institutions and service providers often leverage predictive analytics solutions to improve their KYC (Know-Your-Customer), fraud detection, identity verification, risk assessment and personalization services. In a dynamic industry that relies heavily on tailored decision-making and accuracy, big data proves invaluable.
Retail:
High-street and online retailers rely on big data analytics to understand trending and changing customer preferences, optimize stock management, and deliver personalized shopping experiences across many different touchpoints and marketing channels.
Manufacturing:
Big data analytics carry significant influence in manufacturing, distribution and logistics too. As facilities and warehouses are integral across numerous global supply chains, buyers and suppliers can cultivate more efficient supply chain management overall. Additionally, big data can be used to inform route planning software, predictive maintenance schedules, reduce service downtime and improve operational efficiency.
Big data use cases vary exponentially across a broad spectrum of industries, and are not exclusively limited to those listed above. Securing systems is an ongoing decision to be factored in to mitigate against increasing threats across every sector. This is also the case for firms aiming to embolden security attacks and breaches from any third-party.
Future Trends in Big Data Analytics Decision-Making
It’s wise to assume that new developments and technologies will influence future big data analytics applications and development. AI is accelerating rapidly and its continued convergence with big data analytics looks poised to propel predictive analytics capabilities to new heights. The AI market itself is projected to reach a valuation of $826 billion by the end of 2030, so it’s fair to say that investment in this space is unlikely to slow down.
Furthermore, as IoT devices proliferate and become more accessible, edge computing and real-time collaboration will become increasingly important in big data analytics’ evolution.
Central to these developments is ethical data use. Organizations will need to be mindful of using, maintaining and dispensing data ethically, as there is growing distrust among consumers of how their data is stored and handled. Maintaining ethical standards as far as their data collection, disbursement and deletion will prove instrumental in preserving trust among their customers as well as their brand reputation.
Big data analytics solutions have become essential tools for organizations seeking to thrive in today’s data-driven business environment. By using the power of advanced analytics, machine learning and AI, businesses can unlock hidden insights, make more informed decisions, and gain a competitive edge. As the field continues to evolve, organizations that effectively harness big data analytics will be well-positioned to innovate, adapt and succeed in an increasingly complex and data-rich world.