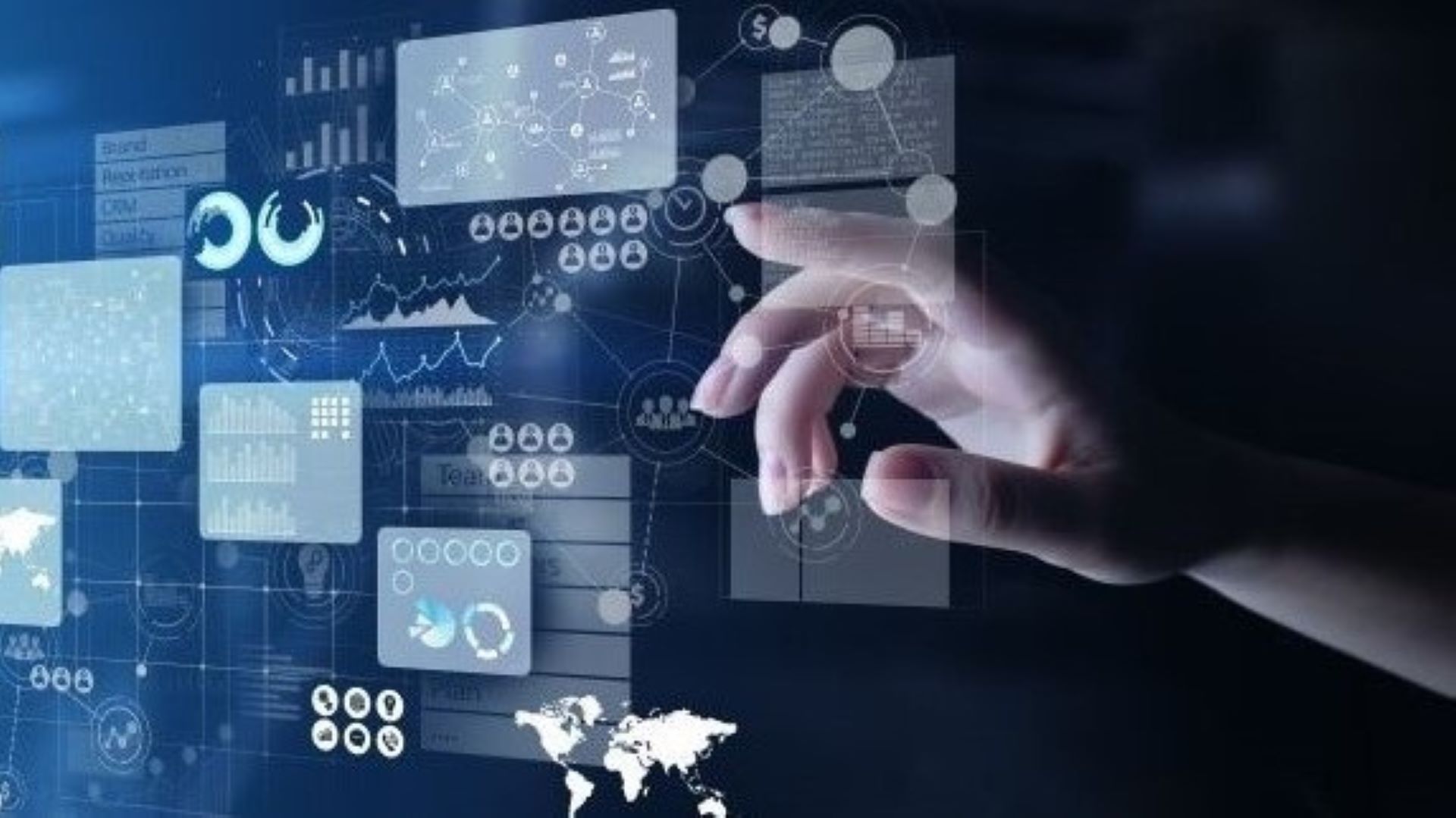
Around 2012, “data science” first dazzled the business world. Commentators glorified the value of big data, comparing it to gold and oil. Technologists celebrated the downfall of HiPPOs (Highly Paid Person’s Opinions) and the rise of “data-driven” decision making.
In reality, most of today’s business leaders rely on false, misleading or unverified analytics for these “data-driven” decisions. Reproducibility, the quality that differentiates data science from pretty graph making, was somehow lost when enterprises took up the banner of big data.
Reproducibility is the principle that a scientist shares not only their conclusion, but the entire process that led them to it. The conclusion is considered reliable only if other scientists can reproduce the process step by step and get the same results (or similar ones).
Despite the obvious risks, the issue of reproducibility isn’t on the radar for most businesses. Their executives routinely make multimillion-dollar decisions based on fictitious insights without realizing it. To put the “science” back in data science, digital leaders must institute workflows and cultural norms that enshrine reproducibility.
The Reproducibility Crisis
The scale of the reproducibility problem in enterprise data science hasn’t been quantified, but the numbers from academia are instructive. In a 2015 study, 270 researchers teamed up to reproduce 98 original psychology papers from academic journals. Only 39% of their replication attempts were successful. Moreover, a 2016 survey by Nature, a peer-reviewed natural sciences journal, found that 70% of biology researchers were unable to reproduce the findings of their peers, and 60% couldn’t replicate their own results.
If scientific analyses by highly trained PhDs are unreproducible two-thirds of the time, why would analyses by enterprise data science teams be any more reliable?
The consequences of unreproducible data can be significant, as a customer of my company, DataChat, learned before connecting with us. This Fortune 100 enterprise had a dashboard that displayed and continuously refreshed key performance indicators (KPIs). During widespread tech layoffs in 2022, the company let go of the people who maintained this dashboard. Two things went wrong.
First, the company couldn’t figure out how to add new KPIs to the dashboard because none of the workflows behind it were documented. Second, when the remaining data scientists finally reverse-engineered the workflows, they found a bug in the code. Every leader at this multibillion-dollar company had acted on KPIs that felt good but were wrong. Had someone attempted to reproduce these analytics before they were first published in a dashboard, the company could have avoided this costly situation.
Reenthroning Reproducibility
Typically, businesspeople debate the meaning of charts and the course of action they recommend. Few question the charts themselves. Once those charts appear on a slide deck, they go unquestioned. Under immense pressure to hit deadlines and quarterly targets, everyone runs with the unverified insights.
So, how does an organization change data processes and culture to champion reproducibility?
- Institute an independent process for verifying analytics: Data scientists either need to manually document every step of their analysis or use a platform that documents steps automatically. Ideally, a data scientist who was not part of the original analysis should attempt to reproduce it using this documentation. A less expensive (but less reliable) option is to have an independent reviewer examine each step of the analysis for issues.
- Welcome open critique and debate: Data analytics can be produced in any organization, but data science only happens when colleagues are free to critique and debate each other’s work, irrespective of job role and seniority. That is how scientists get closer to the truth. In business, however, it’s crucial to include domain experts in this dialogue, which means PhD data scientists need to explain their work in laypeople’s terms and welcome questions.
- Make charts the beginning of the discussion, not the end: Before publishing charts and graphs, data teams must show and revise their work, preferably at the speed of human thought. This doesn’t happen if answering one question from a meeting entails a week of rerunning the analysis with different parameters. Enterprises need documentation and tools that can take analytics from rough draft to final in one meeting. The aim is to produce and reproduce insights while they’re still fresh and relevant.
Taking Nothing at Face Value
Overall, I encourage digital leaders to question every chart and graph. Always ask how the insight was produced and whether it has been reproduced or verified. Be most skeptical of the data that “feels” right or validates your assumptions.
Data science has not lived up to its hype, because enterprises left out the science part. Let’s bring it back. If we don’t take reproducibility seriously, we won’t make data-driven decisions.