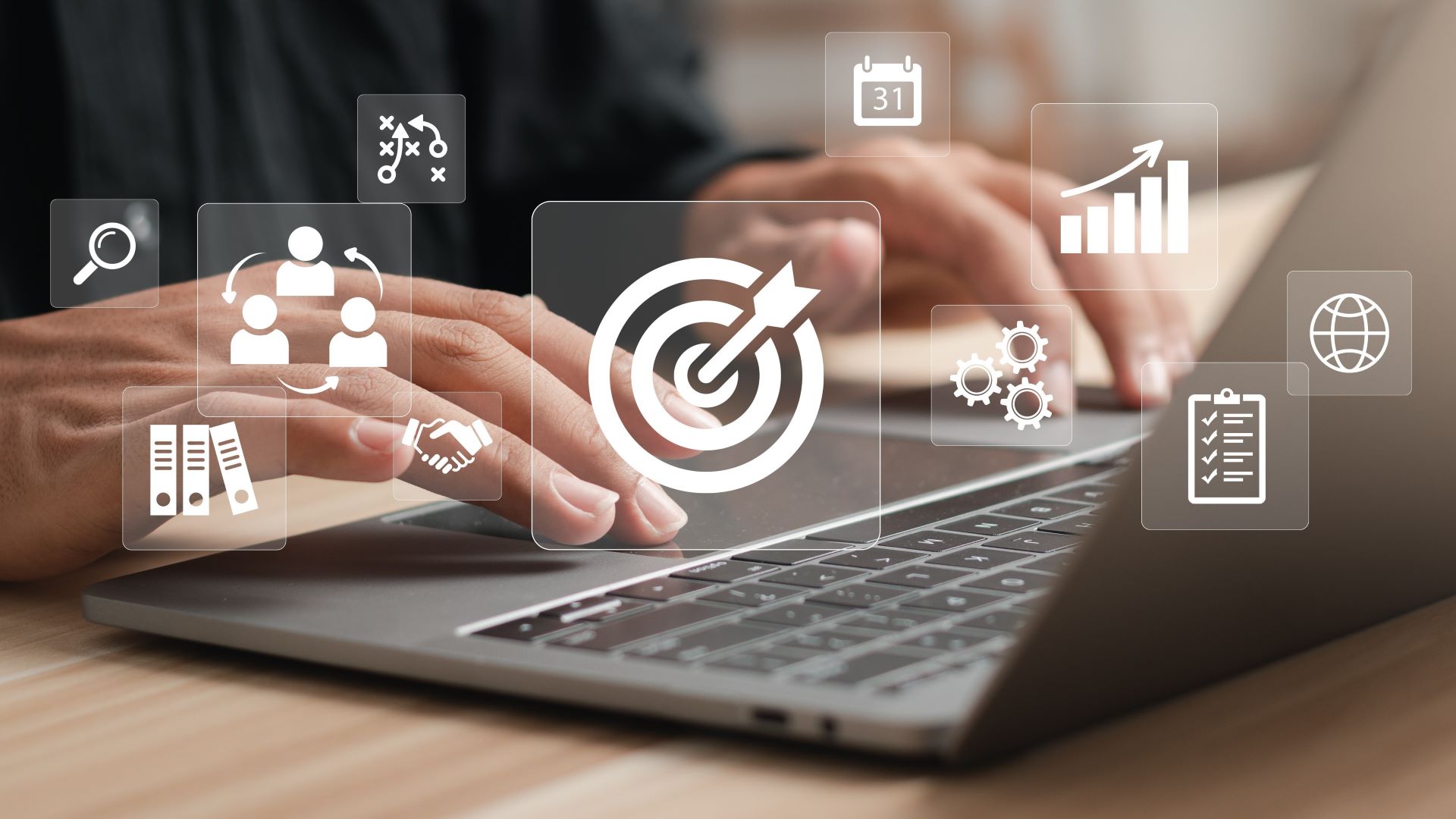
Enterprises have quickly recognized the power of generative AI to uncover new ideas and increase both developer and non-developer productivity, but pushing sensitive and proprietary data into publicly hosted large language models (LLMs) creates significant risks in security, privacy and governance. Businesses need an approach that addresses these risks before they can start to see any benefit from incorporating these powerful new technologies into their digital transformation strategies.
As IDC notes, enterprises have legitimate concerns that LLMs may ‘learn’ from their prompts and disclose that information to other businesses that enter similar prompts. Businesses also worry that any sensitive data they share may be stored online and exposed to hackers or accidentally made public.
That makes feeding data and prompts into publicly hosted LLMs a nonstarter for most enterprises, especially those operating in regulated spaces. So how can enterprises extract value from LLMs while sufficiently mitigating the risks?
Work Within Your Existing Security and Governance Perimeter
Instead of sending your data out to an LLM, bring the LLM to your data. This is the model most enterprises will use to balance the need for innovation with the importance of keeping customer PII and other sensitive data secure. Most large businesses already maintain a strong security and governance boundary around their data, and they should host and deploy LLMs within that protected environment. This allows data teams to further develop and customize the LLM, and employees to interact with it, all within the organization’s existing security perimeter.
You can’t have a strong AI strategy without a strong data strategy to begin with. That means eliminating silos and providing simple, consistent policies that allow teams to access the data they need, all within a strong security and governance posture. The end goal is to have actionable, trustworthy data that can be accessed easily to use with an LLM within a secure and governed environment.
Build Domain-Specific LLMs
LLMs trained on the entire web present more than just privacy challenges. They’re prone to “hallucinations” and other inaccuracies, and can also reproduce biases and generate offensive responses that create further risk. Moreover, foundational LLMs have not been exposed to your organizations’ internal systems and data, meaning they can’t answer questions specific to your business, your customers, and possibly even your industry.
The answer is to extend and customize a model to make it smart about your own business. While hosted models like ChatGPT have gotten most of the attention, there is a long and growing list of LLMs that enterprises can download, customize, and use behind the firewall — including open-source models like StarCoder from Hugging Face and StableLM from StabilityAI. Tuning a foundational model on the entire web requires vast amounts of data and computing power, but as IDC notes, “once a generative model is trained, it can be ‘fine-tuned’ for a particular content domain with much less data.”
An LLM doesn’t need to be vast to be useful. “Garbage in, garbage out” is true for any AI model, and enterprises should customize models using internal data they know they can trust and will provide the insights they need. Your employees probably don’t need to ask your LLM how to make a quiche, or for Father’s Day gift ideas. But they may want to ask about sales in the Northwest region, or the benefits a particular customer’s contract includes. Those answers will come from tuning the LLM on your own data in a secure and governed environment.
In addition to higher quality results, optimizing LLMs for your organization can also help reduce resource needs. Smaller models targeting specific use cases in the enterprise tend to require less compute power and smaller memory sizes than models built for general purpose use cases, or a large variety of enterprise use cases across different verticals and industries. Making LLMs more targeted for use cases in your organization will help you run LLMs in a more cost effective, and efficient way.
Surface Unstructured Data for Multimodal AI
Tuning a model on your internal systems and data requires access to all the information that may be useful for that purpose, and much of this will be stored in formats besides text. About 80% of the world’s data is unstructured, including company data such as emails, images, contracts and training videos.
That requires technologies like natural language processing to extract information from unstructured sources and make it available to your data scientists, so they can build and train multimodal AI models that can spot relationships between different types of data and surface these insights for your business.
Proceed Deliberately, but Cautiously
This is a fast-moving area and businesses must use caution with whatever approach they take to generative AI. That means reading the fine print about the models and services you use and working with reputable vendors that offer explicit guarantees about the models they provide. But it’s an area where companies cannot afford to stand still, and every business should be exploring how AI can disrupt their industries. There’s a balance that must be had between risk and reward, and by bringing generative AI models close to your data and working within your existing security perimeter, you’re more likely to reap the opportunities that this new technology brings.