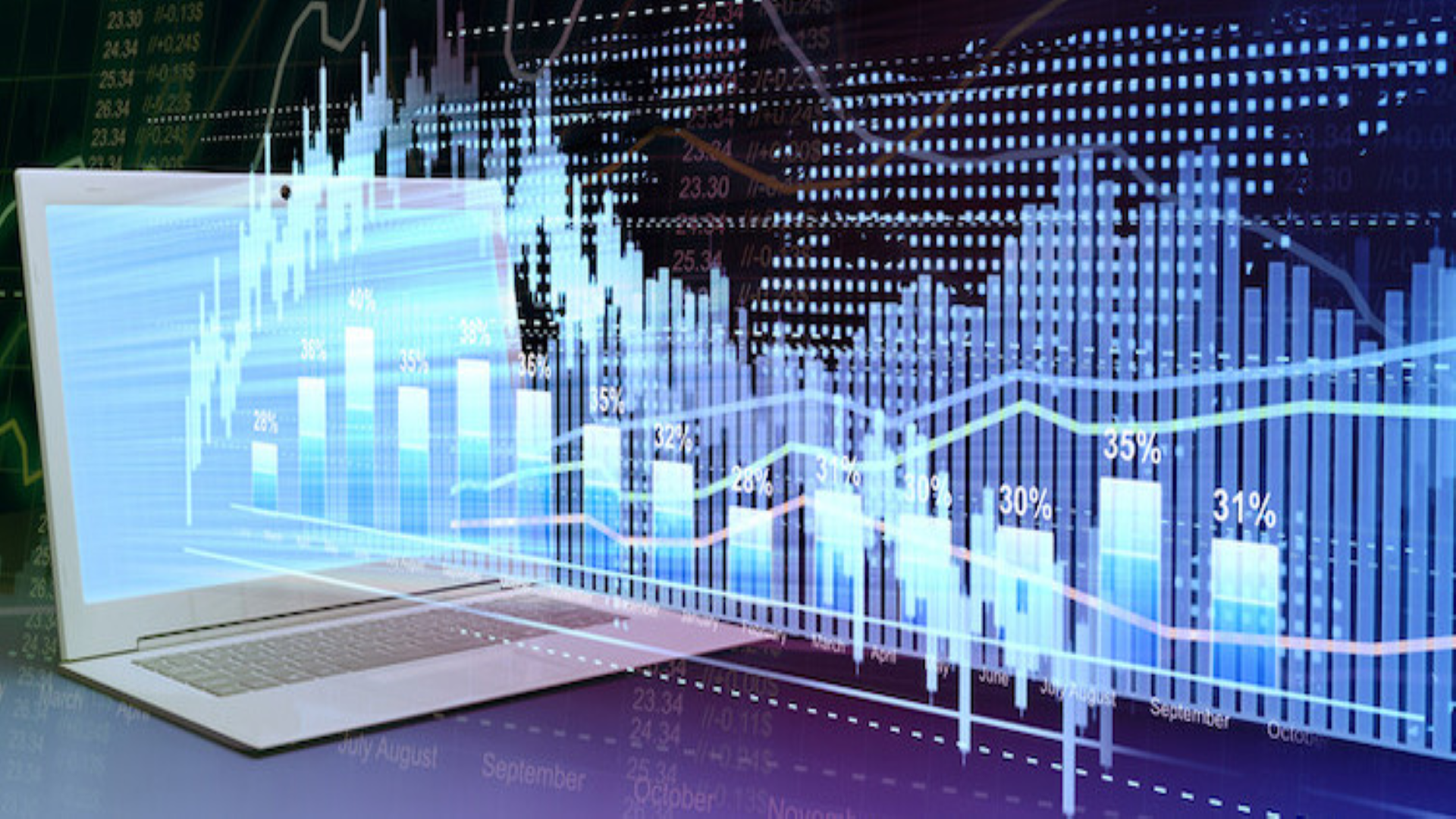
The volume of data and the sophistication of analytical tools to crunch that data has never been greater. And yet, some companies still struggle with getting the needed insights from that data. What is going wrong?
The truth is that likely nothing is going wrong with the technology, the data or the people–on their own. The disconnect sneaks in when different groups–such as Analytics and Line of Business (LOB) executive businesspeople–try and work together while holding very different objectives, understandings and procedures.
The resolution? Companies need an internal interpreter, and such a role already exists. It’s called a data analyst and that approach is the perfect bridge between data scientists and LOB business folk.
To be fair, it may not always be that simple. For one thing, different companies define different data titles differently.
At its core, this disconnect speaks to a basic–and understandable–misunderstanding about what LOBs can realistically expect from data science teams.
Product leaders think in terms of the insights they need to solve for business users for their LOB users. As they should. The data team looks at the data they have and look for what insights they can extract.
If everyone is lucky, both groups get what they need and everyone is happy. But many data groups don’t have a thorough understanding of the business mechanisms, which means they may be fuzzy about the specific insights that the LOB team needs/wants.
On the flip side, the LOB users and their leaders often are fuzzy about the data the company has and what kinds of insights can reasonably be discovered.
In theory, the ideal fix is to train data people into the intricacies of the various key business units and to also train LOB leaders in the minutia of data analysis. I think we can all quickly agree that neither is likely to happen and nor is it even the ideal approach. It’s a nice world you want to live in, but it’s not the one you actually live in.
The much better resolution is to use–or hire and then use–data analysts, who are trained to act as an interface between business and data analysis.
So, you hired a data science team. What’s next? The team you hired are not writing new algorithm approaches. They know what random decision forests are and they are excellent at tuning hyperparameters. But how well do they know the insides of all of your key business lines?
Here’s what you cannot do. Expect to give the data science teams petabytes of data and say “Now tell me how to make more money.” You need to first know if you even have the data that is correlated to the desired and needed answers.
This brings us back to the critical role of the data analyst. Data science teams are notoriously disinterested in the business. Data analysts, however, typically understand the business pretty intimately. The sad truth is that an insufficient number of companies understand the role of the data analyst and they, therefore, fail to use data analysts in useful ways.
Finding experienced and well-trained data scientists is challenging today, but the data scientists that you can find are going to be really technical people. It behooves you to invest in a really good translator.