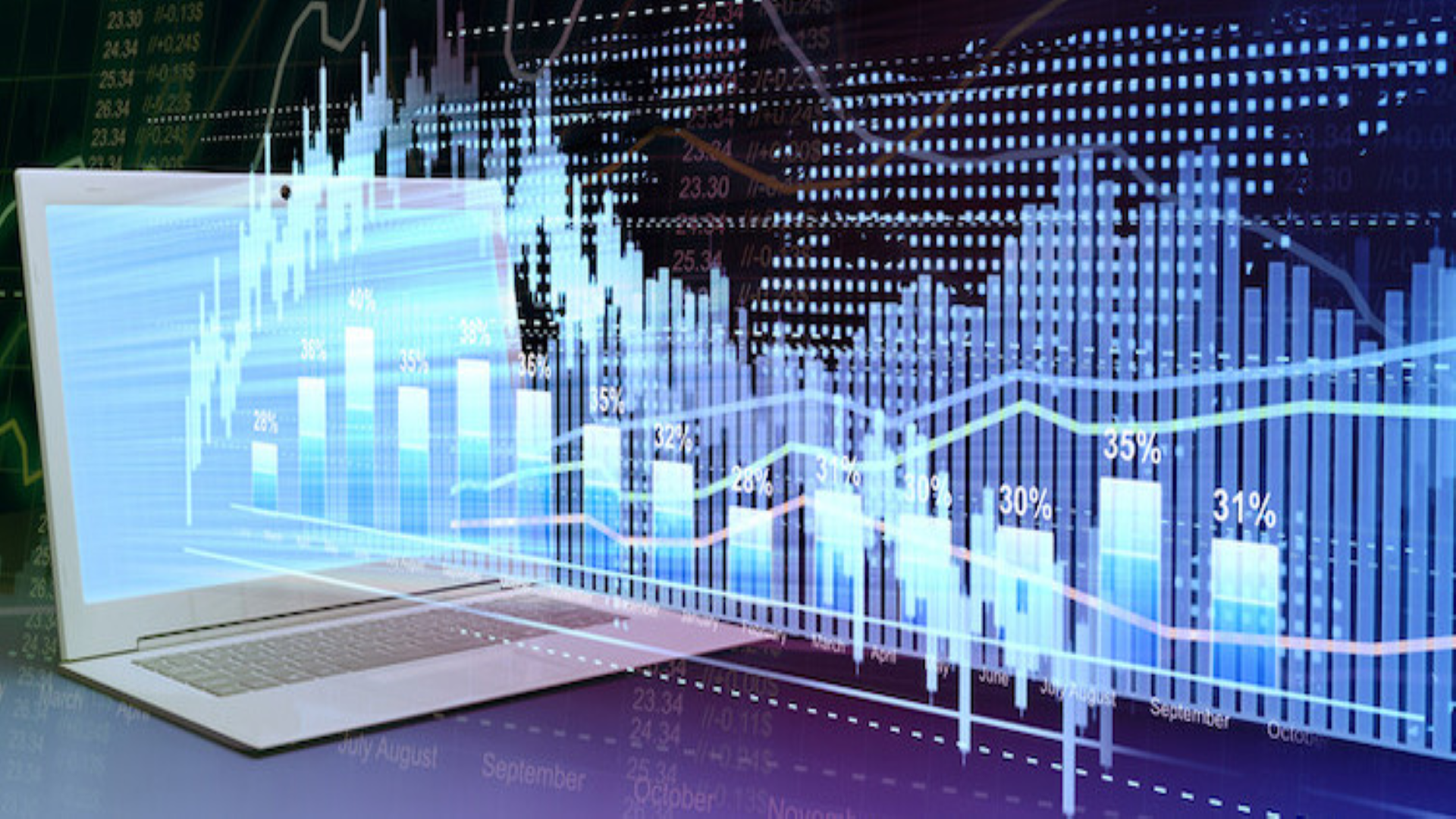
By now, enterprise leaders understand that AI and automation technologies are only as powerful as the data fueling them. Yet, many struggle to grasp just how critical high-quality, user-friendly data is to the success of these technologies. What steps can enterprises take to ensure their data provides accurate, actionable outputs that drive meaningful results?
Despite significant investments in AI and automation – 72% of enterprises have adopted AI for at least one business function – setbacks often arise not from the technology itself but from data quality issues. Without clean, comprehensive data, automation and AI efforts may falter, yielding unreliable insights and flawed recommendations. For these systems to succeed, data must be accurate and timely.
Driving Valuable Insights
User-friendly data is more than just a collection of information—it is data that decision-makers across departments can easily understand and act upon. To achieve this, high-quality data must provide sufficient context for accurate interpretation without relying heavily on technical processing.
Consistency is key; eliminating conflicting records ensures outputs remain reliable. For instance, in financial services, inconsistent transaction data might trigger unnecessary fraud alerts or miss real threats, impacting both security and customer trust.
Timeliness is equally important, as decisions require data that reflect current realities. In health care for example, poor data, such as outdated or incomplete patient history, could lead to inaccurate treatment recommendations.
Most importantly, accuracy underpins everything: Data must correctly represent real-world conditions to deliver trustworthy insights. In retail, for example, inaccurate sales data could cause automation systems to reorder inventory incorrectly, leading to stockouts or overstock situations.
By upholding these principles—consistency, timeliness and accuracy—businesses across industries can ensure data empowers better decision-making and outcomes.
Example: Automated Customer Support Responses
Consider a scenario where a company uses an AI-driven automation system to manage customer support queries. The system prioritizes and categorizes incoming questions based on historical customer data, enabling quick, automated responses for common issues.
If this system lacks complete or accurate data on past queries, it may misclassify incoming requests or offer irrelevant responses. This could lead to dissatisfied customers and, ultimately, damage the brand’s reputation. However, with high-quality, complete data, the system can provide context-aware responses that address customer needs more effectively, streamlining support operations and enhancing customer satisfaction.
For instance, suppose the system recognizes an accurate pattern in common queries related to a new product feature. It might proactively offer relevant resources, anticipate follow-up questions and reduce response time, leading to a more efficient and user-friendly experience.
Ensuring Data Reliability Through Governance
In addition to attaining high-quality data, enterprises must maintain it as well. Business leaders must incorporate robust governance practices, including:
• Data stewardship to monitor and maintain data quality.
• Automated validation processes to detect errors and correct them in real-time.
• Data lineage tracking to trace data origins and understand transformations.
By addressing data quality proactively, enterprises can generate actionable insights and foster trust in their AI and automation systems. Clean, structured data creates a virtuous cycle: Accurate inputs yield meaningful outputs, which in turn drive better decisions.
Quality Data and Sustained Automation Success
Investing in data quality delivers benefits far beyond improved AI and automation performance. It streamlines operations, minimizes risks and strengthens organizations’ competitive positions through data-driven strategies. High-quality data is more than a prerequisite for AI success—it’s the foundation of real business value.