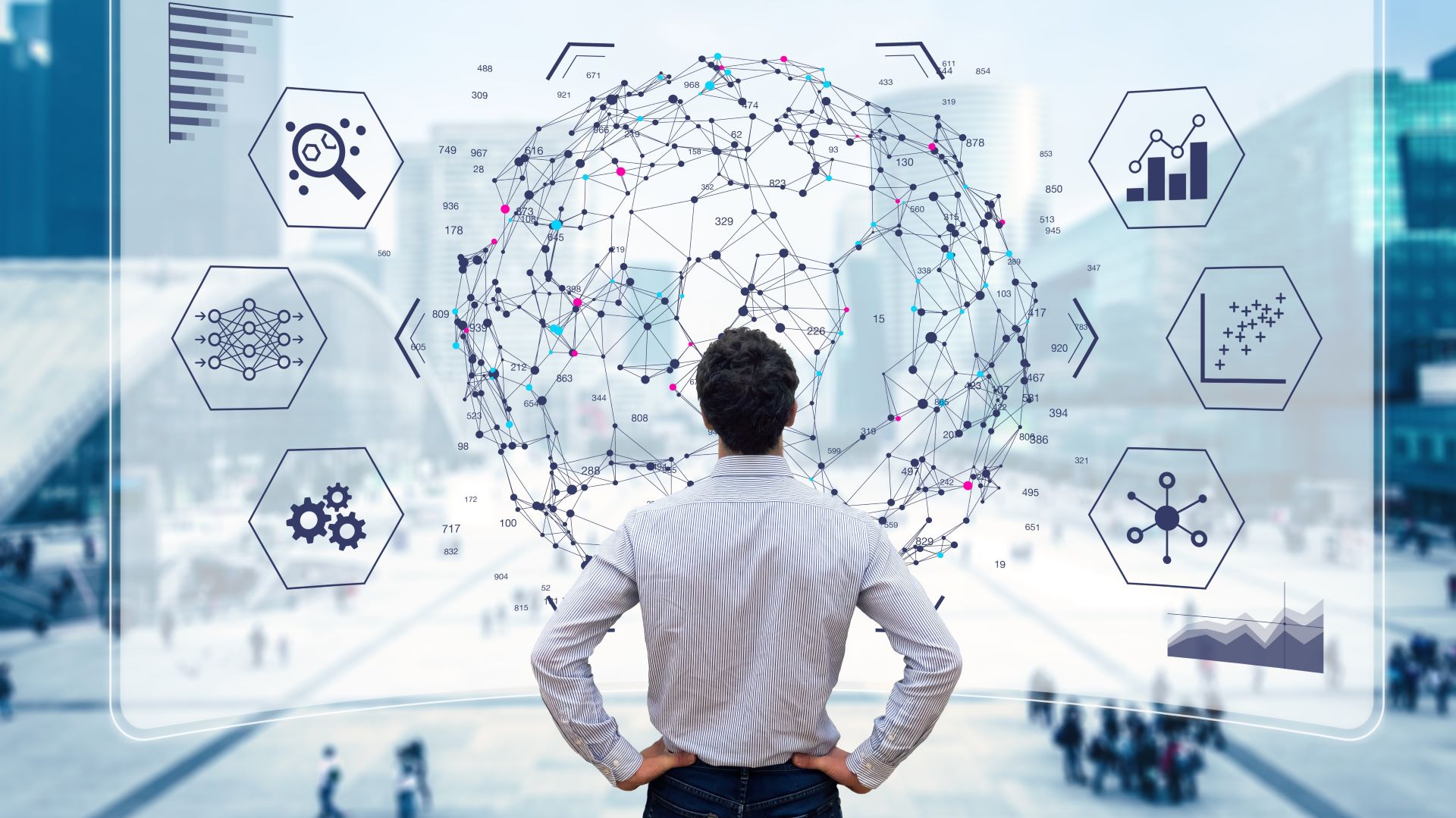
Jared Diamond‘s book, “Collapse: How Societies Choose to Fail or Succeed,” details how civilizations crumbled due to their inability to adapt to environmental challenges, and many of the lessons of Diamond’s analysis boil down to the timeless importance of foresight, adaptability and resource management in the face of transformative change. There are critical lessons for today’s businesses in these lessons.
As artificial intelligence promises to revolutionize industries, many companies are unwittingly steering themselves toward a modern-day collapse by neglecting the critical foundation of data readiness. This situation mirrors the societal downfalls explored in Collapse: Today’s businesses risk obsolescence by failing to cultivate the data ecosystems necessary for AI adoption.
The parallels between ancient societal collapses and the potential downfall of unprepared companies in the AI age are striking, and companies with the foresight to have taken data readiness seriously dare to adapt to the AI-driven hyper-automated business world rapidly on the way, and have the capabilities in place to manage data and digital transformation effectively.
Today, those companies that have invested in robust data infrastructure and quality management are well-positioned to reap AI-driven productivity benefits. Conversely, those grappling with poor data quality and organization face competitive challenges, missed potential and substantial investments in working down their data debt. The tale of two data realities will accelerate as the gap between the data-ready and the data-neglected widens over the next few years.
Poor data quality is already costing organizations and the nation considerably. According to a survey conducted by Precisely in collaboration with the Center for Applied AI and Business Analytics at Drexel University’s LeBow College of Business, only 12% of organizations report their data is of sufficient quality and accessibility to implement AI effectively. With all of the talk around AI adoption, that is a staggeringly low percentage.
Further, that survey also found that 67% of organizations don’t completely trust the data they currently rely upon for decision-making. Surprisingly, that figure didn’t improve year over year, up from 55% in 2023. I suspect that’s likely not a sign that data quality has degraded that much year over year.
It’s most likely a reflection that organizations are finally looking at the state of their data quality and don’t like what they see. The fact is bolstered by the finding that 64% of respondents identified data quality as their top data integrity challenge, up from 50% in 2023, and 77% of respondents rated the quality of their data as average or worse, compared to 66% in the previous year.
Those findings indicate a hefty lift for most organizations regarding AI data preparation, including data collection and labeling. It’s already proving costly. According to a 2020 survey by Trifecta, up to one-third of AI initiatives fail due to poor data quality, rendering these investments ineffective. Further, that survey found that 75% of C-Suite executives don’t trust the quality of their data.
Models trained on inaccurate, incomplete and low-quality data caused misinformed business decisions, impacting organizations’ global annual revenue by 6%, or $406 million on average. And poor-quality data can lead to a 20% decrease in productivity and a 30% increase in costs. Insufficient data costs organizations an average of $12.9 million per year, according to a Vanson Bourne report commissioned by Fivetran, a cloud-based data integration platform provider.
Many organizations struggle with the quality and clean data to maximize AI and digital transformation. There are several interconnected reasons. First, enterprises collect data from so many different sources with inconsistent standards, and these various sources are often of dubious accuracy. This data is usually siloed within departments, and there are so many disparate applications that integration and standardization across departments are challenging. Legacy systems also pose data availability challenges, such as lacking modern API access.
Most enterprises also lack a data governance strategy consisting of a data governance framework, data ownership/accountability, data quality management, integration and a way to monitor and continuously improve data management and not invest the time and other resources necessary for the level of data preparation adequate for effective AI implementation.
This leads to a lack of adequate labeling, which makes machine learning training challenging. Most organizations have failed to implement such data governance that leads to effective data quality management. There’s also an absence of experts available to hire to manage and implement data governance frameworks. All of this leads to data quality issues that surface as inaccurate and inconsistent data, incomplete data, and plenty of irrelevant and biased data.
Companies that are behind in their AI readiness data preparation must improve their data quality. And that should start by conducting a comprehensive data audit to assess the current state of their information assets. That includes evaluating data completeness, consistency, accuracy and freshness across their systems.
Businesses can then focus on cleansing and standardizing their data, removing duplicates, filling in missing values and ensuring consistent formats throughout their datasets. Organizations must also prioritize implementing robust data governance policies and establishing clear data usage, retention and sharing guidelines that align with relevant legal standards.
As organizations continue to invest in AI and digital transformation, maintaining the quality of input data remains a critical factor in determining their success and reliability. The reality is that poor data quality will likely lead to significant financial losses, decreased productivity, potential reputational damage, and for some businesses, failure. However, as in Jared Diamond’s book Collapse, many of those business failures will be caused by the choice of inaction.