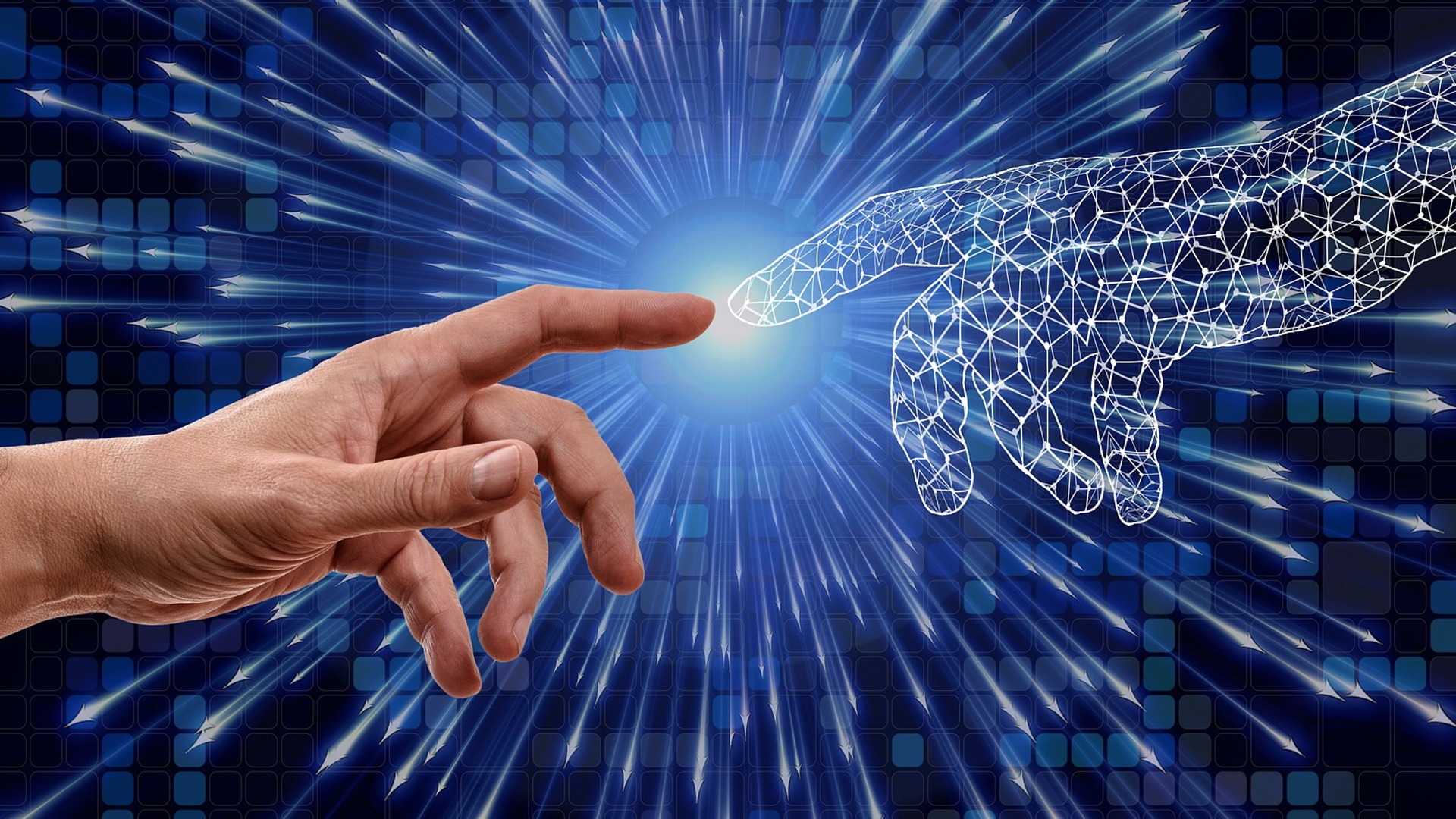
In the past month alone, AI has been accused of “creating a new colonial world order” and credited with ending car crashes, diagnosing cancer and even saving the planet.
The point is that AI is having a massive impact on us every day, both as individuals and as a society. There is so much pressure on businesses to differentiate from their competitors and to operate as efficiently as possible, and those goals are often powered by AI. The benefits are obvious, but the potential downsides can no longer be ignored. The stakes are too high.
Companies are beginning to recognize how challenging it is to achieve true AI maturity and are increasingly making appropriate investments in collecting the immense amounts of data and conducting the continual training of their AI algorithms required to meet that challenge. Many try to manage this entirely on their own, but there are many issues that they commonly face. The most common, and potentially the most dangerous, is the bias of their data.
We as human beings all struggle with bias every day, whether conscious or unconscious. Conscious bias is a learned stereotype or belief system of which a person is aware. Unconscious bias, also called implicit bias, is a set of learned stereotypes of which the holder is unaware. Businesses certainly understand the need to eliminate biases in their hiring practices and employee culture, but unfortunately they often overlook or misunderstand the presence of that same bias in their AI-powered products.
Every developer has conscious and unconscious biases that feed into their initial development, and when that flows into their data collection efforts, it can set a dangerous precedent. Bad data has the potential to cause AI to make biased decisions that can actively harm the people and populations most vulnerable and in need:
- In financial services, “A.I. bias caused 80% of black mortgage applicants to be denied.”
- In healthcare, a Harvard study shared, “Algorithms in health care technology don’t simply reflect back social inequities but may ultimately exacerbate them. What does this mean in practice, how does it manifest, and how can it be counteracted?”
Path to Eliminating Bias in AI
Because AI solutions and users are constantly evolving, there is no single fix to the problem of bias in AI. It is a fundamental issue for any AI program – something you must plan, budget and resource for early in your design and development process. Like AI itself, the testing and refinement process never ends, but it does get better over time with discipline and dedication.
Here’s what you need in order to eliminate bias from your AI program.
You need more data: In many cases, companies work with less data than they need when they develop an AI algorithm. More training data means more learning for the algorithm. Early or smaller sample sizes make it difficult to identify trends and make accurate correlations. Why can’t companies collect the right amount of data? Because doing it right means sufficiently representing all attributes of your customer base. Teaching a machine to learn, think, act and respond not just like a human, but better than a human, requires massive amounts of training data across numerous potential scenarios. This applies to the baseline sets of training data that you collect, and to the ongoing collection of data for your algorithm to learn and adjust. This is a time-consuming process, especially at scale, and one that internal teams struggle to accomplish on their own.
You need more diverse data: More important than just data volume is the proper representation of your desired dataset. Failure to collect a sufficient number of data points for select criteria can quickly result in sampling bias. This is often noticed in a demographic context, but it covers a variety of cases. An individual can interpret one piece of data in multiple ways depending on a number of factors from ethnicity, gender, nativity and more; what one person calls soccer, another calls futbol, and both could be correct or incorrect depending on the application. You need a 360-degree view of your use case to apply the proper labels to each data point. Thus, a diverse perspective is key.
There are many highly-publicized instances where lack of this holistic perspective has become apparent, such as when facial recognition systems fail to identify people of certain skin tones or speech recognition systems fail to comprehend people with speech impediments or specific regional accents. To the consumer, this feels frustrating at best and discriminatory at worst; however, in all but the most egregious cases, it is not an intentional slight but is instead reflective of the fundamental differences inherent in analyzing different types of data and user input. Mature enterprises are those that have both implemented a mechanism to regularly measure such bias and have established partnerships with external data providers to correct this bias through targeted collection of additional data.
You need humans in the loop: More novel algorithms and burdensome regulations cannot fix a problem that is so inherently human – only humans can, and it’s critical that we do. The best way to eliminate bias in AI is to keep humans in the loop. Bias is tough to spot in a QA lab; it takes a large and diverse set of data and testers, and the makeup of those resources needs to be able to flex and grow alongside your evolving products and the changing behavior of your users.
Ethical and accessible AI systems cannot be effectively trained and tested with internal employees, beta programs or off-the-shelf datasets. Such approaches simply cannot capture the breadth of human characteristics and behaviors necessary to adequately support a diverse user base. Companies at the forefront of AI adoption have learned that the only way to do this reliably and efficiently is to leverage a real-world community of individuals representative of the true end user. More than a decade ago, enterprises began to recognize the need to move to the cloud, and today, the leading enterprises are those who recognize the need to move the crowd.
An AI system can’t yet adequately validate another AI system. Only people are uniquely qualified to find biases, glitches and sources of friction in a customer journey, application or business process. The sooner your business embraces that reality, the sooner you can create a truly next generation customer experience.