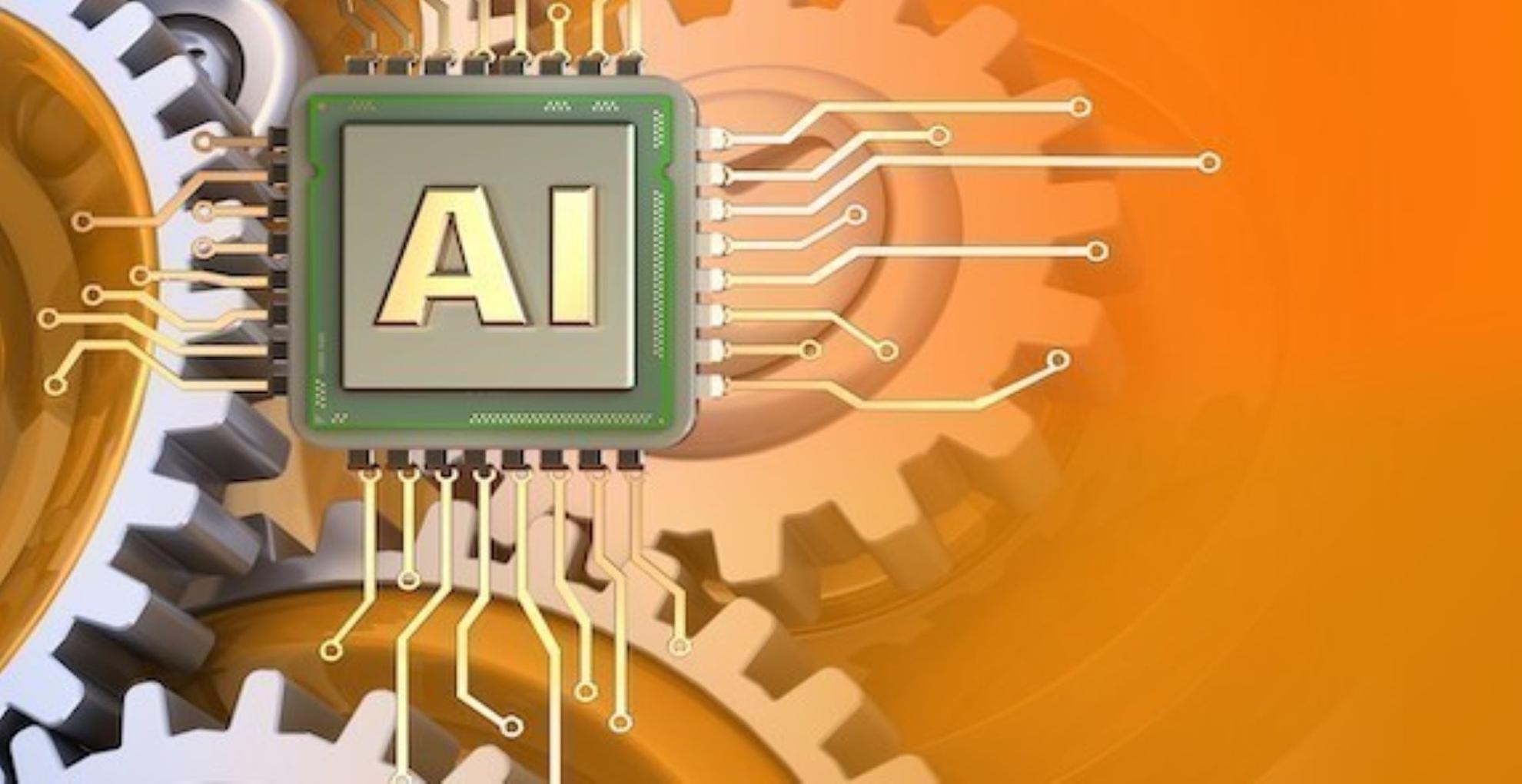
Today, we find ourselves in the midst of Industry 4.0, characterized by the fusion of digital, physical and biological worlds through advanced technologies such as the Internet of Things (IoT), artificial intelligence (AI), and big data analytics. This new era has given rise to smart factories, where machines can communicate with each other and make autonomous decisions to optimize production processes.
Introducing Agentic AI in Manufacturing
The latest frontier in this evolution is the emergence of agentic AI, a progressive technology that combines autonomous decision-making with real-time adaptability. Unlike traditional automation, agentic AI enhances efficiency, reduces costs and fosters sustainable practices, making it indispensable for smart factories.
Agentic AI is reshaping manufacturing processes in several key areas. One of the most significant applications is in predictive maintenance. Traditional maintenance models are reactive, addressing failures after they occur. In contrast, agentic AI enables predictive maintenance, where systems monitor machinery in real-time, identifying signs of wear or potential failure before they disrupt production.
For inventory management, agentic AI systems use real-time data and demand forecasts to optimize stock levels, ensuring the availability of raw materials while avoiding overstocking. Autonomy here reduces carrying costs and improves supply chain efficiency, allowing manufacturers to maintain lean inventories while meeting production schedules seamlessly.
In robotic assembly lines, agentic AI enables dynamic task allocation and real-time adaptability. Unlike traditional robots that follow pre-programmed instructions, AI-powered robots learn from their environment and adjust to changing tasks on the fly. This significantly reduces errors, optimizes resource usage, and enables scalability in production, making them a cornerstone of smart factory operations.
It’s important to understand that the implementation of intelligent automation and agentic AI in manufacturing is not about replacing human capital. Instead, it’s about reallocating human resources to other critical areas of manufacturing planning, business operations, analysis, operations, and reporting functions.
Examples For Varying Industries
Let’s consider some industry-specific examples to illustrate how intelligent automation and agentic AI are being applied:
In the aerospace industry, automated decisioning powered by agentic AI is changing aircraft maintenance and manufacturing. For instance, predictive maintenance algorithms can analyze data from sensors throughout an aircraft to predict when components are likely to fail, allowing for proactive maintenance and minimizing downtime. In manufacturing, AI-powered robots can perform intricate assembly tasks with high precision, improving quality and reducing production time.
The automotive industry is leveraging intelligent automation for everything from supply chain optimization to autonomous vehicle development. Agentic AI is being used to optimize production schedules, manage inventory, and even design new vehicle models. In assembly lines, collaborative robots (cobots) work alongside human workers, handling repetitive tasks while humans focus on more complex assembly operations.
In the construction industry, intelligent automation is improving project planning and execution. AI algorithms can analyze historical project data to provide more accurate cost and time estimates. On construction sites, autonomous vehicles and drones are being used for surveying and material transport, improving safety and efficiency.
The military and defense sector is utilizing intelligent automation and agentic AI for logistics, training, and even combat operations. AI-powered systems can optimize supply chains, ensuring that troops have the necessary equipment and supplies when and where they need them. In training, virtual and augmented reality systems powered by AI provide realistic simulations for soldiers, improving readiness and reducing training costs.
The Use of Digital Twins
A key component in the implementation of automated decisioning across these industries is the use of digital twins. A digital twin is a virtual representation of a physical object or system, updated in real-time using data from sensors in the physical world. In manufacturing, digital twins of production lines or entire factories allow for real-time monitoring, predictive maintenance, and optimization of operations.
For example, in aerospace manufacturing, a digital twin of an aircraft engine can be used to simulate different operating conditions, predict wear and tear, and optimize maintenance schedules. In automotive production, a digital twin of the assembly line can help identify bottlenecks, optimize workflows, and even test new production configurations virtually before implementing them in the physical world.
The integration of digital twins with agentic AI takes this concept even further. AI algorithms can analyze the vast amounts of data generated by digital twins to make autonomous decisions and optimizations. For instance, an AI system could use data from a digital twin of a manufacturing plant to automatically adjust production parameters in real-time, optimizing for factors such as energy efficiency, output quality, and equipment lifespan.
As we look to the future, the potential of intelligent automation and agentic AI in manufacturing seems boundless. These technologies are not just improving efficiency and reducing costs; they’re enabling new levels of customization, sustainability, and innovation. By 2025, it’s predicted that AI-powered automation could save manufacturers up to 25% of their operational costs.
However, the successful implementation of these technologies requires careful planning and a holistic approach. Companies cannot delay advancing their digital journeys, including AI adoption. Early adopters are already creating competitive advantages, and the gap with the laggards looks set to grow. A successful program requires firms to address many elements of a digital and analytics transformation: identify the business case, set up the right data ecosystem, build or buy appropriate AI tools, and adapt workflow processes, capabilities, and culture.
The evolution of intelligent automation, culminating in the current era of agentic AI, represents a true shift in manufacturing and supply chain management. Through embracing these technologies, manufacturers can improve their operational efficiency and decision-making processes while also freeing up their human workforce to focus on more strategic, creative and value-adding activities. The successful integration of intelligent automation and agentic AI will likely be a key differentiator in the competitive landscape of manufacturing, driving innovation, sustainability, and growth across industries.