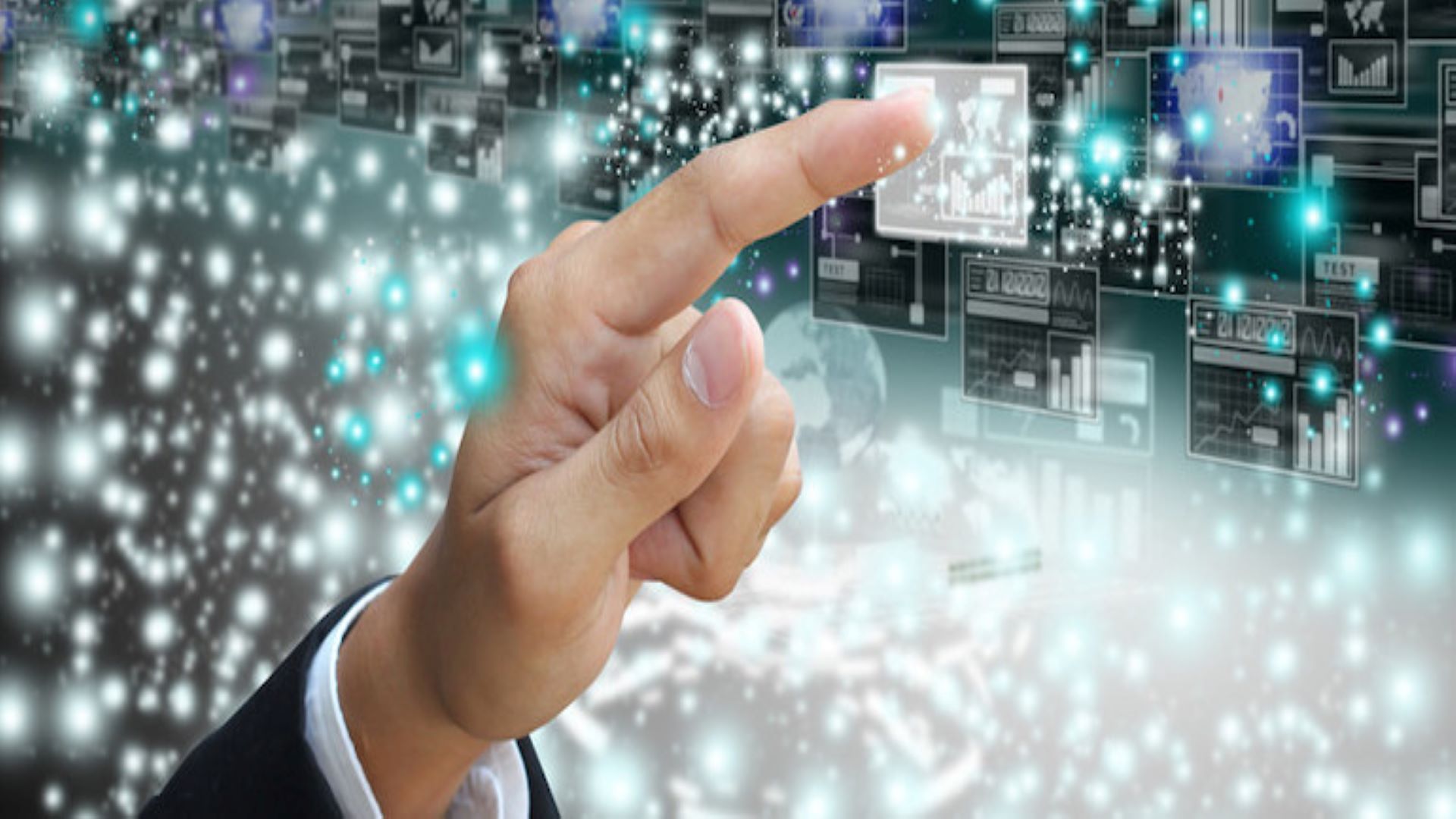
As of 2021, Gartner reported an annual $12.9 million spent on poor-quality data, which has only been accelerated by the world’s shift to a digitally-driven society since social-distancing began. Businesses have experienced a great waste of funds through digitization of sources, as well as organizing and hunting for information within their systems.
Beyond the loss of revenue, bad data – or the lack of it – ultimately results in poor decision-making and, unintentionally, uneducated business assessments. Beyond simply collecting data, it has to be actionable to add any value to businesses, and in order for that to happen, the data must be readily accessible.
In this article, we’re going to discuss how deep learning can increase data’s structure, accessibility and accuracy, all while avoiding massive losses in revenue and productivity.
Manual Data Entry Is Hindering Your Productivity
Companies often work with physical copies of data, in the forms of scanned documents, PDFs or images. In fact, there are an estimated 2.5 trillion PDF documents globally, yet, businesses still struggle to automate the extraction of accurate and relevant data from paper and digital-based documentation.
Difficulties involving this process usually result in unavailable data or poor productivity, neither of which are suitable for the increasingly digital-driven world we’re in. Not to mention the potential implications this same challenge can have on compliance and the millions it can cost an organization breaking data regulations.
While manual data entry may seem like a good method for converting sensitive documents into actionable data at a low scale, it does allow for increased chances of human error as the number of documents increase. It can evolve into increased costs for the hours spent on data entry, and furthermore, the potential costs of resolving human errors and not keeping up with data regulations, such as GDPR or CCPA.
Although these tasks can become daunting, they are also a huge opportunity for automation, as long as we can capture the data correctly and easily, and maintain data accessibility and accuracy. But how do we do that?
Understanding The Benefits of Machine Learning
Over the past few decades, machine learning (ML) has been an integral technology for ramping up everything we do. It’s been a goal since ML’s creation to utilize data and algorithms to emulate human learning processes, gradually improving its accuracy. It’s no surprise that these advanced technologies have been so widely adopted in the midst of the digital revolution.
We’ve reached a point of no return as the amount of data generated each day is expected to reach 463 exabytes across the world by 2025. This only emphasizes the necessity and urgency of creating processes that can support technological evolutions on par with the exacerbated growth of data.
Maintaining, organizing and analyzing data is becoming more and more a technological step. For example, data extraction APIs have the ability to increase digital competitiveness by making data more accurate, structured and accessible. In this context, data portability gains a major role, ensuring that users are protected from locking in their data in “silos” or “walled gardens” that are potentially discordant with one another, hence causing complications – with data backups for example – in the future.
Thankfully, there are a few best practices to consider when contemplating utilizing the power of machine learning for data portability and accessibility at an organizational level:
-
- Prescribing and utilizing proper algorithms – In order to fulfill data scientists’ needs and support their research, data must come with strict and detailed technical standards. All transferring and/or exporting of data needs to be performed in a way that organizations can maintain compliance with user data regulations and deliver insights for the business. For example, document processing used to extract PII (Personal Identified Information) from a PDF needed for HR purposes can be stored in the same database as the data extracted from a receipt (in terms of amounts paid and dates), as long as each data set is stored in a predefined data structure suitable for the specific data. Various functions can be easily automated with the appropriate algorithms.
- Designing applications capable of using those algorithms – Businesses will continue to gather data from different file types, and to streamline extraction they can train their algorithms to provide more accurate results over time. On top of that, the quantity of file/data types should increase to continue expanding on the use case.
- Security must be top of mind – The data your company generates and collects is crucial and highly private. As the journey of incorporating ML into gathering important data progresses, it’s important to prioritize security; from maintaining closed doors for personal identifiable information (PPI) to ensuring data stored can be retrieved or deleted as needed for compliance reasons.
- Training models – In order to train ML models, they need high-quality data, and most importantly this data needs to be stored in the format that the information will be processed in. This is vital, as the implications of the insights gathered and delivered to stakeholders depend on it. Furthermore, if the data fed to the algorithm is high quality, the algorithm will more accurately identify and deliver specific insights for the business.
Ultimately, data is only useful if it’s accessible. If your data is unrecognizable and unusable by a machine, you cannot automate any processes. While the process can be complicated in the beginning, when following the steps above, the benefits outweigh the time and money spent setting it up. The business will experience, among many other benefits, the acceleration of insight delivery for faster decision making, reduction of overall costs of manual data extraction, provision of higher productivity by facilitating faster data retrieval, and improvement in data accuracy through ML/AI and end-user experience.
Embracing Technology: The Cornucopia of Data
The evolution of technology has provided us with the opportunity to let tech-driven automation complete the more tedious, yet important admin tasks, so that humans can focus on developing the valuable insights gained from data.
No matter how much data businesses collect, the real power lies in the ability to make decisions from company information and data that is easily and swiftly accessible, along with the confidence that said data will be correct. We’re all aware that most work-specific processes begin with a single document. Now, what we do with that document has evolved: we have been on a path to move away from manual data input, into controlling data. Control of your data guarantees that the information your business is using for expansion, decision-making and customer acquisition is high quality.
It’s time to embrace the value brought on by this structure. After all, data is only valuable if it is actionable. Along your digital transformation journey, keep in mind that the higher quantity of accurate data you share with a machine learning model, the better the results you will secure.