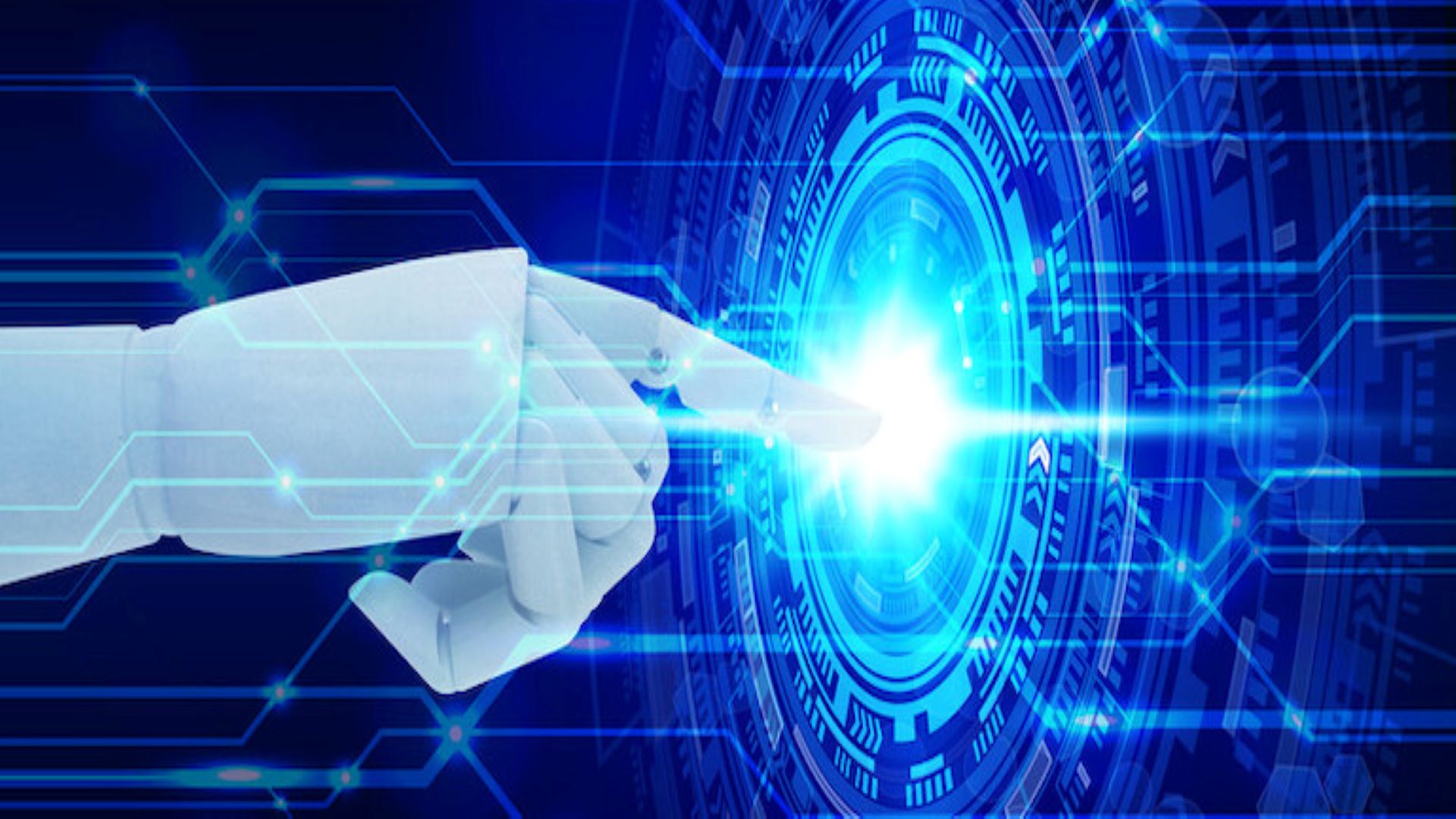
Enterprises have been investing heavily into artificial intelligence and machine learning and will continue to invest in the years ahead in these technologies. According to Allied Market Research, worldwide enterprise AI investments was just shy of $5 billion in 2018 but will reach $53 billion by 2026. That’s an annual growth rate of roughly 35% and far exceeds other areas of enterprise technology investments.
“Over the past decade, we’ve been laying the foundations for AI-driven transformation of our technology and data capabilities, so that we can effectively harness and operationalize machine learning and AI so that they aren’t just buzzwords, but have actual business impact everything from our customer experiences down to our bottom line,” says David Kang, SVP and head of data insights at Capital One.
This week, Capital One released a study it commissioned to Forrester Consulting, based on responses from 150 data management decision-makers in North America about their organizations’ machine learning goals, challenges and plans to operationalize their machine learning efforts.
Most Enterprises “Experimenting” with Machine Learning
The survey found that over half of survey respondents reported that their organizations have been developing and releasing applications for one year to two years, but within that timeframe, respondents were still “mostly experimenting” with machine learning. The study concluded that a mature AI/ML strategy does not begin to take shape until about three years or more into their machine learning efforts. The survey found fewer than a quarter of respondents’ to be currently mature.
Of those machine-learning mature enterprises, the Forrester Consulting study found that roughly 75% reported that their “AI platform was their core business platform for delivering value at the departmental level or higher.”
The study also found that as organizations continue to experiment with machine learning, enterprises are focused on increasing efficiencies across their organizations, while also building a foundation for future machine learning success with “IT-heavy data and analytics strategies, focusing on multi-cloud, building a better performance stack, and scaling to changing data demands,” the study concluded.
The study authors also predict that as organizations do increase their machine learning maturity, their strategies will shift with that increased maturity. Currently, the survey found that enterprise data and analytics strategy focuses on multi-cloud (46%), technology performance stack (44%), and building the scale necessary to handle increasing demands (41%) for data.
While 53% of enterprises plan to improve the efficiency of their businesses through machine learning, machine learning goals will quickly adapt. In fact, as machine learning evolves, data and analytics strategies will shift alongside it, with deployment of ML to automate anomaly detection replacing multi-cloud as decision-makers’ top priority in the next one year to three years. Once they lay the foundation and move toward maturity, decision-makers will likely shift their focus to growing revenue and improving CX with their ML applications. Within the next one to three years, the focus will be deploying machine learning to automate anomaly detection (40%), obtain automated and transparent application and infrastructure updates (39%), and meet new regulatory and privacy mandates (39%).
Barriers to Machine Learning Maturity
The report found that there are many barriers enterprises must overcome if they are to successfully operationalize machine learning. The biggest challenges, respondents said, include breaking down departmental data silos (41%), converting academic machine learning models into products that can be deployed (39%), removing silos associated with external data partners (38%) and working with large, diverse, and messy data sets (36%).
Fifty-seven percent of respondents believe silos between data scientists and practitioners inhibit ML deployments. But that is by far not the only challenge enterprises face when it comes to getting the value they seek from machine learning. The survey found that 73% of respondents believe that data transparency, traceability and explain-ability of data flows are challenging.
Finally, despite the challenges enterprises face garnering effective machine learning deployments, to keep C-Suite on-board when it comes to machine learning, data teams are focusing their machine learning efforts on cross-business outcomes. That includes making it easier to move data from one platform to another (65%), ability to track where and how data moves within their organization (63%), and using machine learning to shorten the time to action (59%).
“The takeaways I had are that there’s still tons of promise and tons of excitement for machine learning and artificial intelligence to be integrated into everyday business processes to improve customer experiences and also drive better outcomes for businesses,” says Kang. “Yet, the barriers and the speed bumps to getting that impact are still very much present,” he concludes.