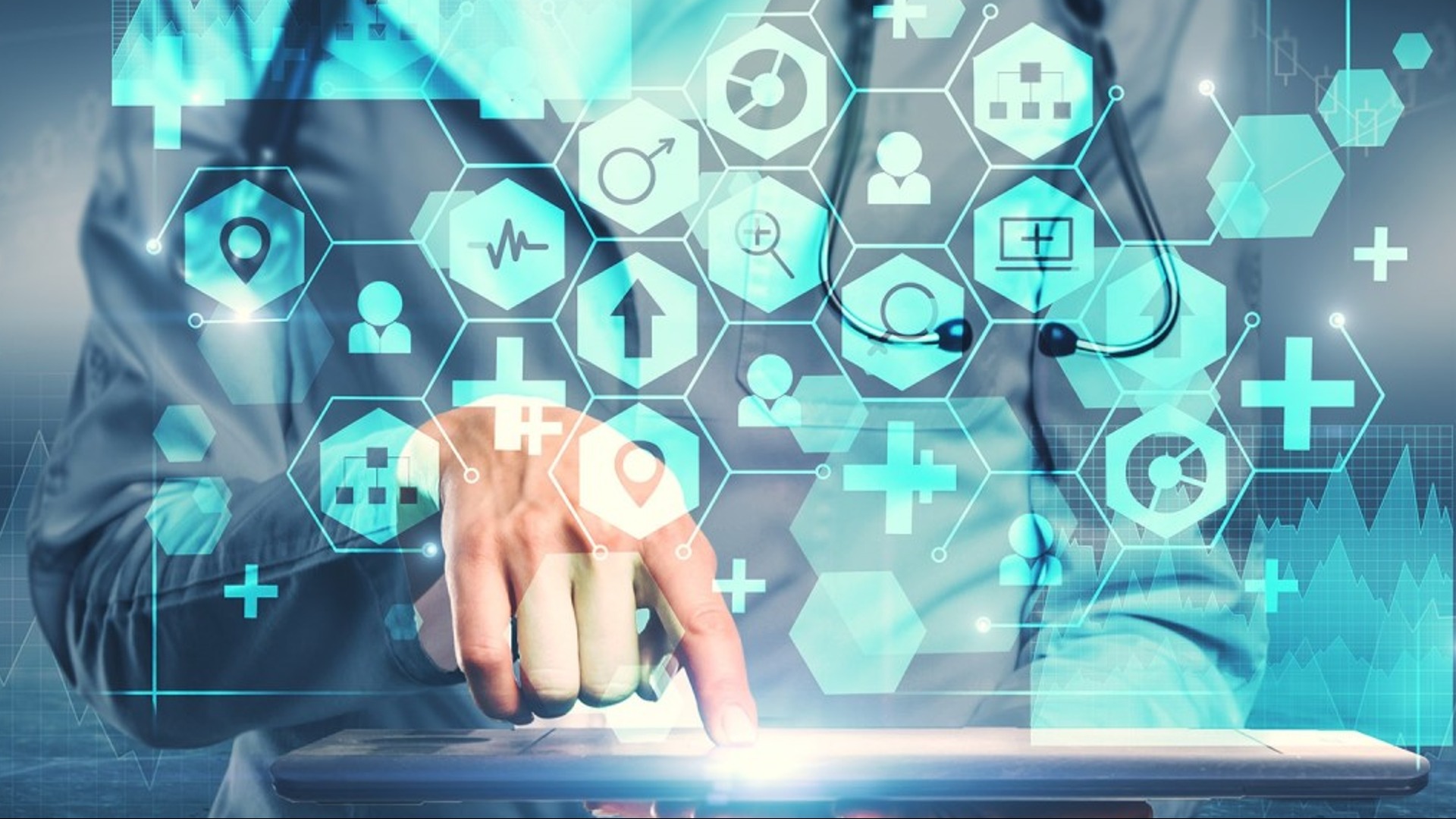
Data integration is a critical challenge that health care organizations must address to provide high-quality care and make informed decisions. Health care data comes from many sources, including electronic health records (EHRs), laboratory systems, imaging systems, billing and claims databases, and wearable devices.
Integrating this disparate data is essential for creating a comprehensive view of patient health, optimizing operations, and supporting advanced analytics. However, the path to effective data integration could be more challenging. This article explores these challenges and how Online Analytical Processing (OLAP) can offer solutions.
The Complex Landscape of Health Care Data Integration
Integrating health care data is a challenge due to the complexity and variability of the data sources involved. To fully understand this landscape, it is essential to delve deeper into the primary obstacles that health care organizations face in achieving seamless data integration.
Data Heterogeneity
Health care data comes in various formats and sources, each with its unique structure and standards. These sources include:
- Electronic Health Records (EHRs):
-
- EHRs are digital versions of patients’ paper charts that store detailed medical histories, diagnoses, treatment plans, immunization dates, allergies, radiology images, and laboratory test results. EHR systems may use different data formats and terminologies, complicating data integration efforts.
- Clinical Notes and Unstructured Data:
-
- Physicians’ notes, discharge summaries, and other narrative forms of data often need to be more structured. Natural language processing (NLP) techniques are required to extract meaningful information from this text data.
- Medical Imaging Systems:
-
- Radiology, pathology and other imaging systems generate large volumes of unstructured data through images and scans. These require specialized storage and integration methods combined with other patient data.
- Laboratory Information Systems (LIS):
-
- Laboratory tests produce structured data with specific coding systems (e.g., LOINC for lab results). Integrating this data with other health care data requires mapping and standardization efforts.
- Billing and Claims Data:
-
- Financial data related to billing, insurance claims, and reimbursements often follow different standards (e.g., ICD diagnosis codes). Integrating this financial data with clinical data is essential for comprehensive health care analytics but is challenging due to differing data structures.
- Wearable Devices and Remote Monitoring:
-
- Wearable health devices and remote monitoring systems generate continuous streams of data related to vital signs, physical activity, and other health metrics. This data must be integrated in real-time with other patient data for effective monitoring and intervention.
Data Volume
Health care organizations generate and collect a large number of data daily. This data volume presents several challenges:
- Storage and Management:
-
- The sheer volume of data requires significant storage capacity and robust data management solutions. Ensuring all data is stored efficiently and accessed when needed is a continuous challenge.
- Data Processing:
-
- Large data volumes necessitate potent data processing capabilities. Integrating and analyzing these large datasets requires advanced computational resources and efficient algorithms to ensure timely insights.
Data Quality and Consistency
Maintaining high data quality is crucial for reliable health care analytics. Key issues include:
- Inconsistent Data Entry:
-
- Variability in how data is entered into systems can lead to consistency. For instance, different clinicians may use different abbreviations or terms for the same condition, complicating data integration.
- Missing or Incomplete Data:
-
- Only complete patient records or missing values can skew analysis and lead to correct conclusions. Ensuring complete and accurate data entry is a significant challenge.
- Data Standardization:
-
- Different systems and data sources often use different coding schemes and formats. Standardizing these codes (e.g., SNOMED CT for clinical terms, ICD for diagnoses) is essential but challenging.
Privacy and Security Concerns
Health care data is susceptible and subject to strict privacy and security regulations. Challenges include:
- Regulatory Compliance:
-
- Compliance with the HIPAA regulations in the United States requires rigorous data protection measures. Ensuring that integrated data systems comply with these regulations is complex and resource-intensive.
- Data Encryption and Access Control:
-
- Protecting data from unauthorized access and breaches involves implementing robust encryption and access control mechanisms. Managing these security measures across integrated data systems adds to the complexity.
Interoperability Issues
Interoperability is a significant challenge:
- Proprietary Systems:
-
- Many health care systems are proprietary and not designed to interact with other systems. More standardization is needed to ensure seamless data exchange.
- Standardization Efforts:
-
- Efforts to standardize data formats and communication protocols (e.g., HL7, FHIR) are ongoing, but adoption could be more consistent. Ensuring that all systems adhere to these standards is challenging.
- Legacy Systems:
-
- Many health care organizations still use legacy systems that are unnecessary to modern data integration technologies. Upgrading or changing them can be expensive and disruptive.
How OLAP Can Help
Online Analytical Processing (OLAP) can play a pivotal role in addressing these data integration challenges by providing a robust framework for organizing, analyzing, and presenting healthcare data in a multidimensional manner. Here’s how OLAP can help:
- Facilitating Data Integration:
-
- OLAP systems are designed to integrate data from multiple sources into a cohesive data warehouse. By transforming and standardizing data, OLAP enables the seamless combination of structured and unstructured data, providing a unified view of information.
- Handling Large Data Volumes:
-
- OLAP technologies are optimized for handling large volumes of data. They allow for efficient analysis of vast datasets, enabling health care organizations to effectively manage and analyze big data.
- Enhancing Data Quality and Consistency:
-
- Through the ETL (Extract, Transform, Load) process, OLAP systems can clean and standardize data before it is loaded into the data warehouse. This standardization ensures that the integrated data is of high quality and consistent, essential for reliable analytics.
- Ensuring Privacy and Security:
-
- OLAP systems incorporate robust security features to protect sensitive health care data. They support access controls, encryption, and auditing capabilities, ensuring data integration processes comply with privacy and security regulations.
- Improving Interoperability:
-
- OLAP systems can leverage data standards and interoperability protocols to facilitate communication between disparate health care systems. By standardizing data formats and ensuring compatibility, OLAP helps overcome interoperability challenges.
Real-World Applications
Several health care organizations have successfully implemented OLAP solutions to tackle data integration challenges:
- Population Health Management:
-
- By integrating data from EHRs, claims, and social determinants of health, OLAP tools help health care providers gain insights into population health trends, identify at-risk groups, and develop targeted intervention strategies.
- Clinical Decision Support:
-
- OLAP systems integrate clinical data from various sources to support real-time decision-making. Physicians can access comprehensive patient profiles, track disease progression, and make informed treatment decisions.
- Operational Efficiency:
-
- Hospitals use OLAP to integrate operational data, such as staffing levels, bed occupancy rates, and equipment utilization. This helps in optimizing resource allocation, reducing costs, and improving patient care delivery.
Conclusion
Data integration in health care is a complex yet essential task for improving patient outcomes, operational efficiency, and overall health care delivery. Online Analytical Processing (OLAP) offers a powerful solution to address the myriad challenges of integrating diverse health care data.
By providing a framework for efficient data integration, OLAP empowers health care organizations to use their data for advanced analytics and better decision-making.