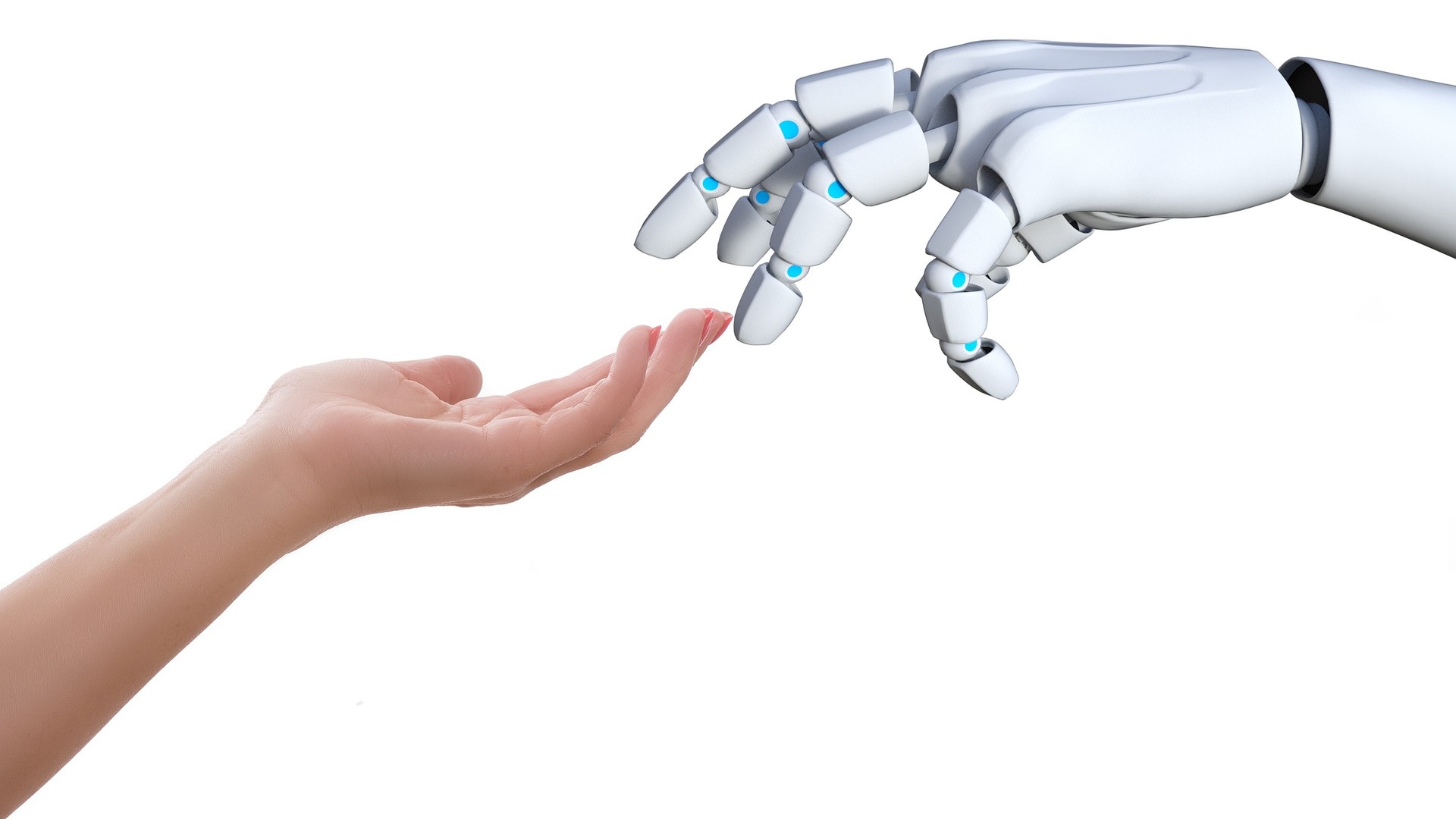
AI is revolutionizing digital twin technology, finally overcoming IoT’s integration challenges and delivering real business value across industries.
Ten years ago, when General Electric unveiled their digital twin technology for aircraft engines, we were on the cusp of an industrial revolution. The idea was compelling: Create virtual replicas of physical assets that could be monitored, analyzed and optimized in real-time. However, as many early IoT enthusiasts discovered, the gap between concept and widespread implementation proved wider than anticipated.
Fast-forward to 2025, and digital twins are experiencing a renaissance, powered by advances in artificial intelligence addressing the challenges that once held the technology back.
The Evolution: From Static Models to Cognitive Replicas
Early digital twins were primarily data visualization tools with basic predictive capabilities. While groundbreaking, GE’s aircraft engine twins required extensive manual configuration and domain expertise to extract actionable insights.
Today’s AI-enabled digital twins are fundamentally different. They’re not just passive virtual representations but active cognitive models that learn, adapt, and even anticipate changes in their physical counterparts without explicit programming.
What’s changed is that modern digital twins don’t just mirror physical systems—they understand them. Machine learning algorithms now identify patterns that human engineers might miss, creating a continuously improving feedback loop.
Breaking Down the Integration Barrier
The hardware-software divide was indeed a critical stumbling block. IoT implementations required rare “full-stack” engineers comfortable with embedded systems and cloud architecture. AI has changed this equation in several ways:
-
Self-configuring sensors: The next generation of IoT devices uses AI at the edge to auto-calibrate and adapt to their environment, requiring far less manual configuration.
-
Unified development platforms: Tools like Siemens’ Xcelerator and Microsoft’s Azure Digital Twins have evolved to bridge the gap between physical modeling and software development, creating environments where domain experts and software engineers can collaborate more effectively.
-
No-code/low-code interfaces allow subject-matter experts to interact with digital twin data without deep programming knowledge directly.
Many organizations report that they spent years trying to find engineers who could work across the full IoT stack. Now, with advanced tools, mechanical engineers can work with AI assistants that translate their domain knowledge into digital twin configurations, cutting implementation time by as much as 70% in some documented cases.
Real-World Impact: Where AI-Enabled Digital Twins Are Delivering Now
Predictive Maintenance That Works
Early predictive maintenance often generated excessive false alarms or missed critical failures. Today’s AI-powered twins have dramatically improved accuracy.
Norwegian oil company Equinor deployed AI-enhanced digital twins across offshore platforms, which reduced unplanned downtime by 68% in 2024, with false positive rates below 3%, numbers that were unimaginable with previous generations of the technology.
Urban Infrastructure Management
Singapore and Barcelona are implementing comprehensive urban digital twins that integrate building systems, traffic patterns, energy usage, and environmental sensors. These systems don’t just monitor, but actively optimize resource allocation based on real-time conditions and predictive modeling.
Singapore’s digital twin anticipated rainfall patterns during a recent storm and automatically adjusted drainage systems, preventing flooding that would have affected thousands of residents.
Health Care Systems Optimization
Hospital networks have deployed facility-wide digital twins that track everything from patient flow to equipment utilization. Mount Sinai Hospital in New York reported a 27% improvement in emergency department throughput after implementing an AI-enabled system that could predict patient surge hours in advance and automatically suggest staffing and resource adjustments.
The Breakthrough Technologies Driving Change
Several specific AI advances have been instrumental in digital twins’ renaissance:
Foundation Models for Anomaly Detection
Large foundation models pre-trained on industrial equipment data can now detect subtle anomalies that would elude traditional rule-based systems. These models transfer knowledge between seemingly unrelated physical systems, identifying patterns that specific-purpose algorithms miss.
Reinforcement Learning for Optimization
Digital twins now employ reinforcement learning algorithms that continuously test different configurations in the virtual environment before applying changes to physical systems. This allows for safe experimentation and optimization without risking operational disruptions.
Multimodal AI Integration
Modern digital twins integrate data from multiple sources—vibration sensors, thermal imaging, audio analysis, and visual inspection—process them through specialized AI models to form a comprehensive understanding of physical assets.
Looking Ahead: The Next Five Years
The actual transformation is just beginning. Here’s what industry experts predict for the near future:
Self-Healing Systems
Digital twins will move beyond prediction to autonomous correction. Early examples include self-healing power grids that can reconfigure to prevent cascading failures, and manufacturing lines that automatically adjust parameters to maintain product quality despite component wear.
Cross-System Optimization
Digital twins of separate systems will increasingly interact with each other, creating networks of virtual models that optimize collectively. Imagine city traffic management systems coordinating with building energy management and public transportation to holistically optimize urban resource usage.
Knowledge Preservation and Transfer
As industries face workforce challenges from retiring experts, AI-enabled digital twins are becoming repositories of institutional knowledge, capturing the insights and intuition of experienced personnel and making them available to new generations of workers.
The Human Element Remains Essential
Despite these advances, the human element remains crucial. The most successful digital twin implementations pair AI capabilities with human expertise.
The goal isn’t to remove people from the equation. Rather, it’s to elevate human decision-making by handling the data complexity that overwhelms traditional analysis. Engineers aren’t being replaced—they’re being empowered.
For organizations that struggled with early IoT implementations, now may be the time to revisit digital twin technology with fresh eyes. The barriers of hardware-software integration and specialized talent requirements have significantly diminished, while the potential business value has only increased.
As we look to the future, it seems the long-promised IoT revolution didn’t fail—it was simply waiting for AI to catch up.