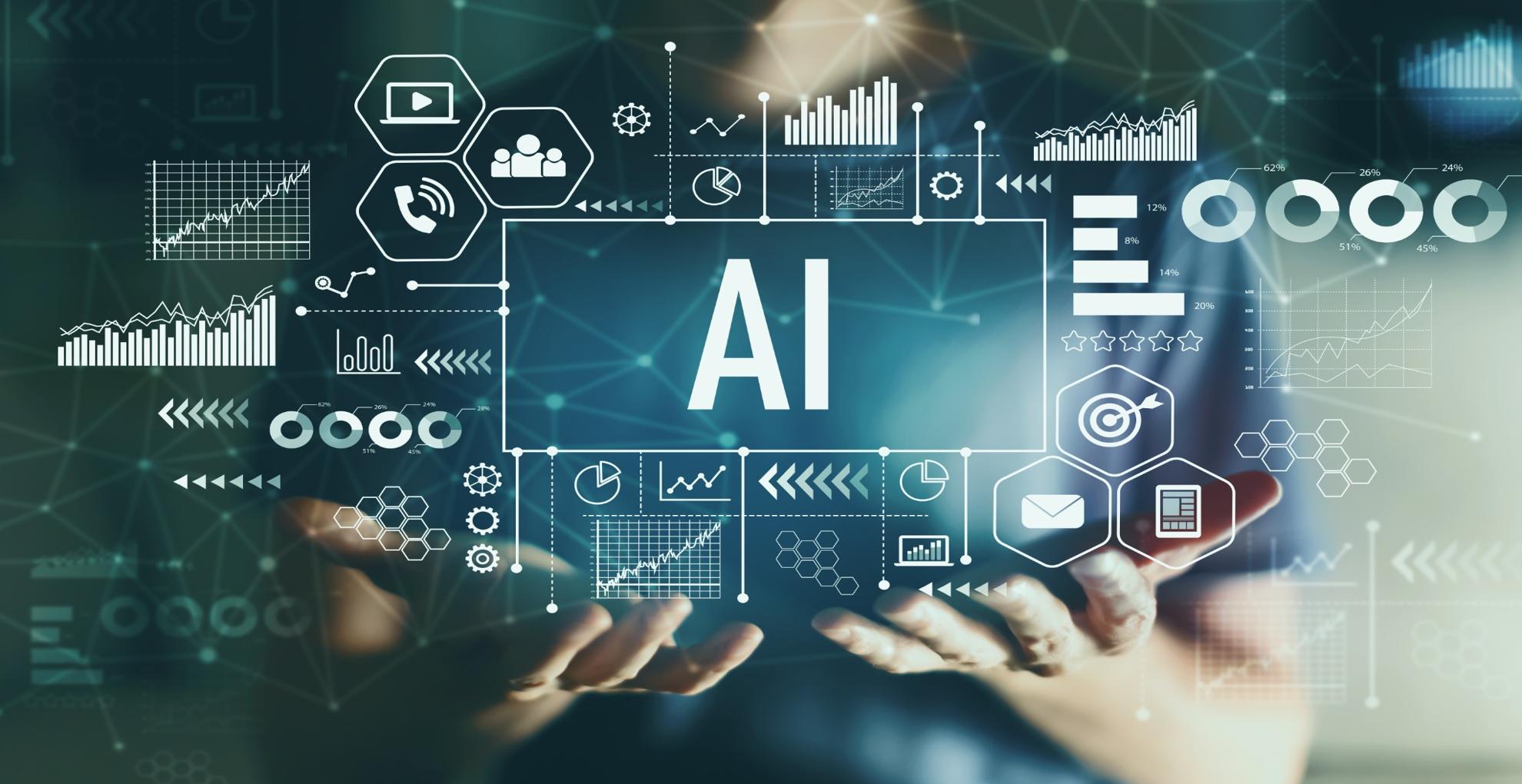
It isn’t a lack of data that plagues today’s decision makers—quite the opposite—it’s an overwhelming surplus. Decision-makers now find themselves buried under a constant avalanche of data, streaming in from countless sources: Internal systems, external feeds, market research, customer behavior analytics, financial reports, operational metrics, etc. Each source offers a different angle and framing, and often a different bias.
Now, with the advent of artificial intelligence (AI), things should be getting simpler and easier. These new tools are almost magical, but what can we trust, how much, and how do we know?
What was once a scarcity problem has transformed into a complexity problem. The very tools designed to aid decision making—business intelligence (BI) and AI platforms—have proliferated over the last decade, and in the case of AI, during the last couple of years.
Initially, they introduced basic data aggregation, then evolved into sophisticated visualization layers known as dashboards. With AI, we have come to expect good brainstorming and data generation in the form of text, images and sound. In theory, this should have ushered in a golden age of insight. In practice, it has fallen short.
Why hasn’t this solved the problem? Because these systems have simply stacked more data atop existing silos. While they present the information more cleanly, they rarely integrate the underlying context that an executive needs to interpret the data meaningfully. Context—understanding why the data matters, how it connects to strategic intent, and what decision it is meant to inform—is the missing link. Without it, dashboards are little more than pretty noise.
The result is a paradox: The more data we have, the longer it takes to extract actual information from it. The decision-making process becomes bogged down, not because there is too little insight, but because there is too much static. Executives are forced to navigate through layers of disjointed inputs, chasing fragments of relevance, often relying on others to translate and interpret what they’re seeing. This elongates the “time-to-information” cycle, stretching it to the point where opportunities are missed and agility is lost. With AI, the trust factor has also arisen to make things even more complicated.
Information. That is an interesting word. What is information to a decision-maker?
To a decision-maker, information is not just data—it is the most essential and strategic asset available. It is the foundation upon which judgments are built, courses of action are determined and resources are allocated. This means information must be trusted, and how can you trust something that is not consistently accurate? In the fast-paced world of business and leadership, every decision incorporates implications of time and cost. Good information reduces uncertainty—sharpens focus, accelerates action and increases the likelihood of successful outcomes. Poor, insufficient, or inaccurate information, on the other hand, leads to delays, missteps and costly inefficiencies.
But how does one gain information that is reliable, relevant and timely?
The only practical and sustainable way to acquire such information is through an interface that not only collects data but also understands and maintains context—context being the unique circumstances, goals and nuances that surround a particular decision-making scenario. Gathering facts is easy. Maintaining relevance and alignment with the decision-maker’s intent is the real challenge. Ensuring that the information generated is accurate is the foundational requirement to build trust.
In traditional business intelligence (BI) and reporting environments, this interface has been the human analyst—someone who interprets the business need, constructs dashboards, builds analytics and compiles reports. This analyst plays the critical role of translator between the world of raw data and the world of actionable insight. Yet, this model, while functional, is limited by time, human bandwidth, and the loss of nuance in translation.
With the advent of probabilistic AI, such as GenAI (neural networks and transformer-based approaches), tremendous power has been added to the ability to generate information. The challenge is that these systems are based on statistical approaches that cannot, by definition, generate accurate answers consistently. In the AI industry, this is known as hallucinations, errors and bias. While very useful for creative endeavors, these tools are generally not trustworthy for decision-making, which is why most organizations avoid using them for mission-critical use cases or decision-making.
Enter the age of deterministic AI, particularly when combined with natural language processing (NLP). In this new paradigm, the interface is no longer a separate human entity—it is the decision-maker themselves. Executives are now empowered to directly interact with systems, using natural language to pose questions, refine scope, shift perspectives and guide exploratory analysis. The AI, in turn, understands and adapts to the evolving context and degree of granularity.
This is more than just automation—it is a transformation of the information-gathering process itself. Executives no longer wait for reports or struggle to extract meaning from complex charts or dashboards. Instead, they conduct a live, iterative, conversational process that mirrors how humans naturally seek insight: Through questions, clarifications and narrative exploration.
The final facet of the contextual framework involves the executive’s ability to evaluate whether the information returned by the AI system can be trusted. Trust, in this context, is not a matter of intuition or belief—it is built on the ability to independently validate and consistently reproduce results. For a deterministic AI system, this means that the same inputs, under the same conditions, should reliably produce the same outputs.
The IT department and executives must have the tools and visibility to trace how conclusions were reached, verify the logic or data underpinning the outcomes, and confirm that the AI behaves predictably across repeated trials. Without this reproducibility and transparency, confidence in the system’s outputs—and thus its practical utility—remains fundamentally limited.
In this environment, information becomes a dynamic and fluid asset—shaped in real time to match the pace and precision of executive thought. The decision maker is no longer just a consumer of data but becomes an active participant in the very structure of insight.