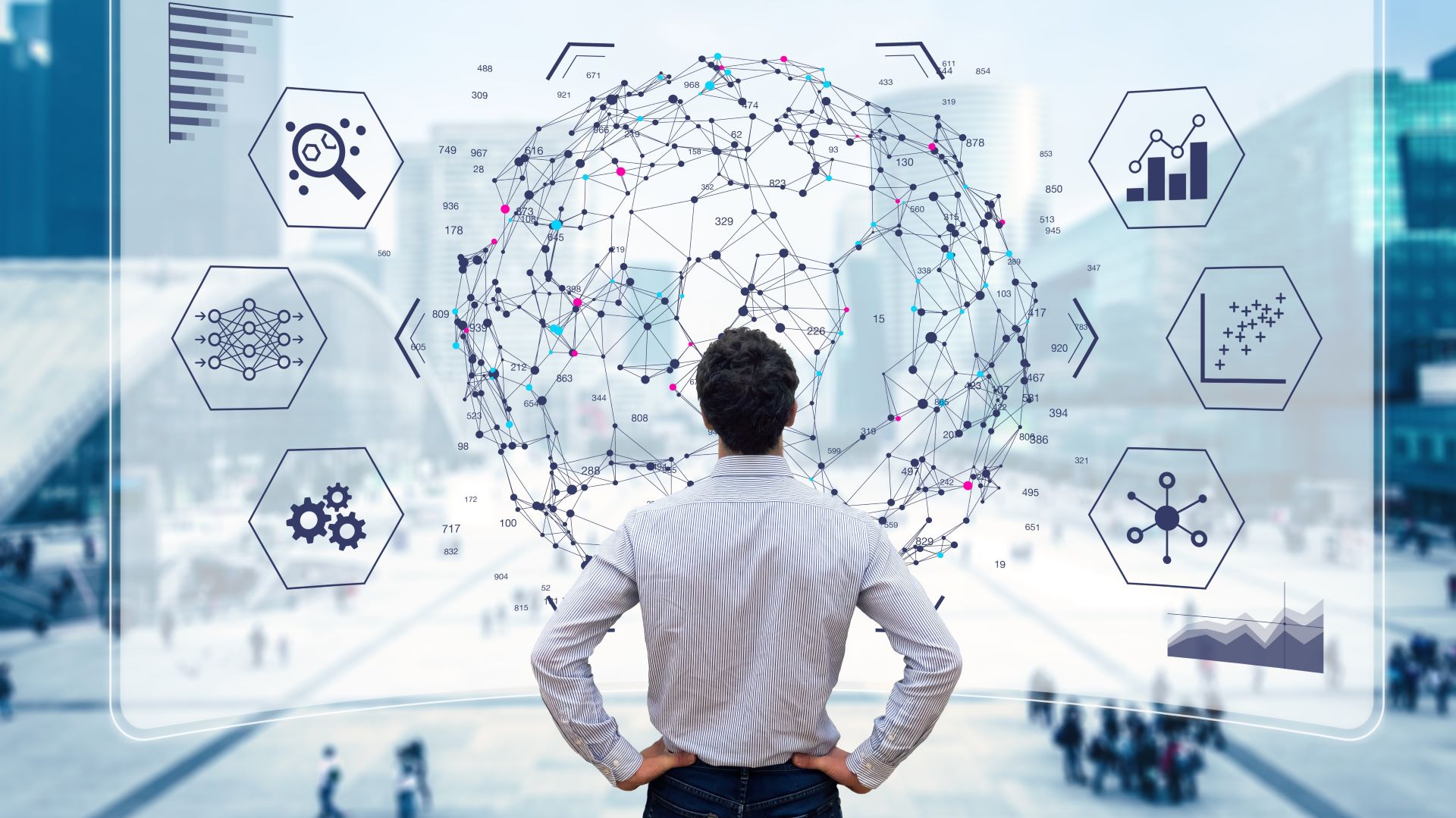
As more data continues to shift toward the cloud, the need to get data in and out of these IT environments at scale is becoming a bigger challenge. IT organizations of all sizes are struggling with getting massive amounts of data in and out of cloud computing environments at unprecedented rates of speed as organizations look to employ analytics in near real time.
The challenge is the data management tools that most organizations relied on in the past to move data are batch oriented. As such, they are not able to keep pace with the need to continuously stream data into a cloud computing environment. New classes of data engineering tools will be required to enable streaming analytics.
For example, Informatica this week launched four different tools for transferring data at high speeds into and out of the Snowflake cloud data platform, including a public preview of a Informatica Snowpipe for Snowflake tool that leverages application programming interfaces (APIs) optimized for data streaming capabilities to ingest and replicate data into a Snowflake environment three times faster than its existing tools.
Expected to be generally available in the second half of this year, Snowpipe for Snowflake simplifies a process that today requires a lot of data engineering expertise to achieve, notes Jitesh Ghai, executive vice president and chief product officer for Informatica.
The need for legacy approaches to batch-oriented data management isn’t going away as much as new uses cases enabled by demand for streaming analytics are becoming more common, notes Ghai. “The difference is these are becoming mission-critical workloads,” he says.
The issue is that as more data than ever moves across wide area networks (WANs) there needs to be more efficient ways to transfer it all, Ghai adds.
Of course, Snowflake is only one of several repositories, also known as cloud data warehouses or data lakes, that organizations might employ. In theory, organizations would prefer to standardize on one single data repository but in the multi-cloud computing era it’s already apparent that most organizations will wind up having multiple repositories. The more repositories there are, the greater the data engineering challenge naturally becomes.
The issue only becomes more aggravated as organizations invest in artificial intelligence (AI). The amount of data needed to train AI models will be extensive, with most of those models employing graphical processor units (GPUs) that are accessed via a cloud service. Those models are also only as accurate as the data used to train them, so there is going to be a lot more emphasis on ensuring best data management practices are maintained.
Unfortunately, data management today in a lot of enterprises is something of a mess. Data sources not only conflict; they are also in some instances completely erroneous. Before that data winds up in an AI model that “hallucinates” because it has been trained using faulty data there need to be a lot more focus in data quality assurance.
In the meantime, there is a growing need for speed when it comes to transferring data. The challenge now is to make sure that the data being transferred is of the highest quality possible.