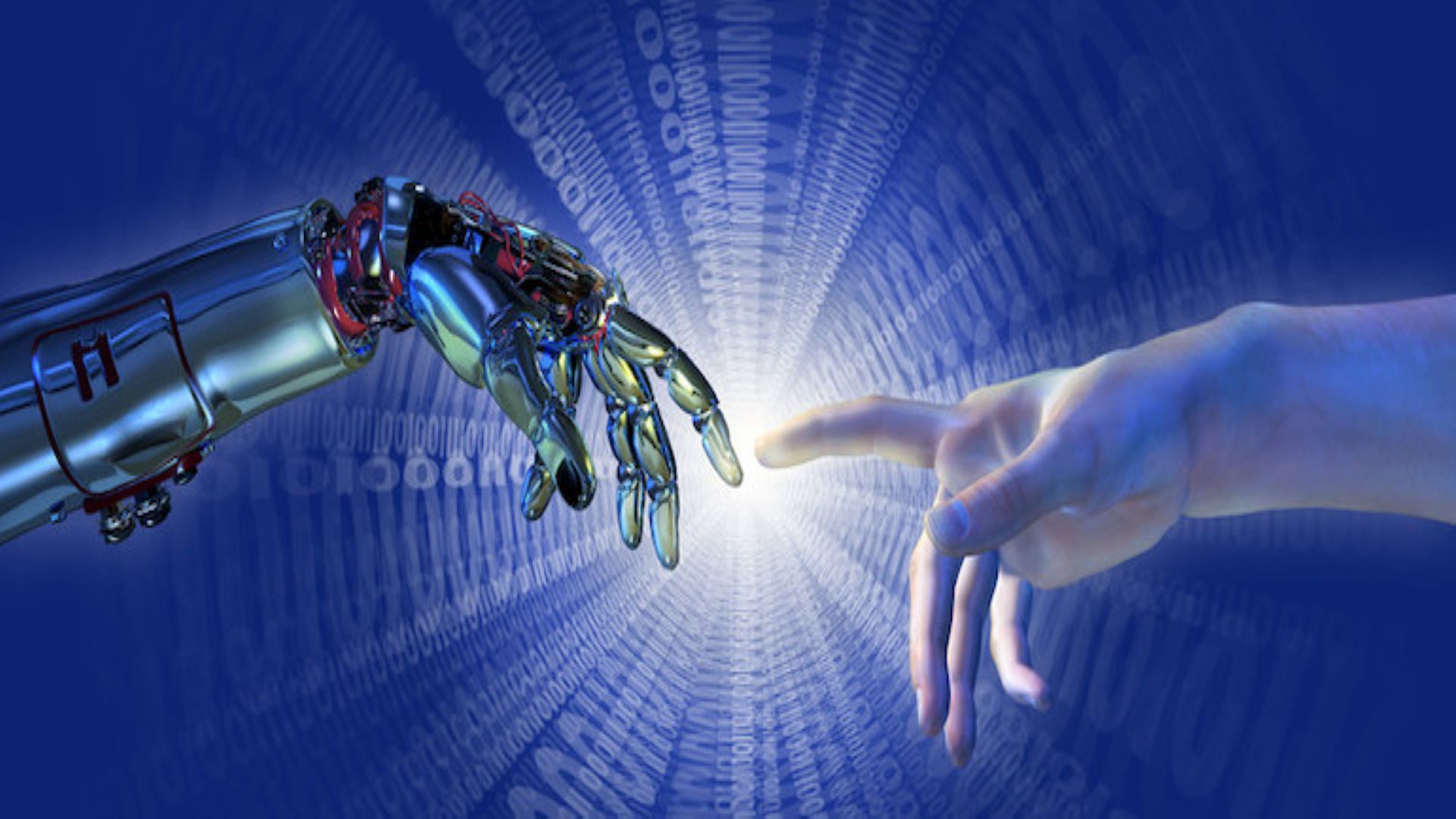
AI ethics are a set of principles and values that, above all, ensure no harm is done. This includes a commitment by everyone involved in a data science project to create models that are safe, inclusive, accurate, fair, explainable and take potential biases into account. This has become a mainstream topic, especially in response to the role technology has played in growing hate crime, destabilizing democracy, radicalizing youth, and scaling up discrimination — all while profiting from it.
In healthcare and life science, Ethical AI and Responsible AI are essential when life-and-death decisions are being automated. Consider a medical device which decides how much insulin or chemotherapy to administer to a patient. If you’re using models trained on data that underrepresented a given ethnicity or race, it can be downright dangerous to people that the models were never optimized for.
This level of responsibility has resulted in broad discussions about the need for data scientists to take a form of the Hippocratic Oath. Perhaps this is something we can expect to see in the next few years, as AI becomes a bigger part of the industry. But even that is not a fix-all solution. So, what are the biggest concerns and how can we address them? For now, it’s a work in progress, but it starts in the development phase.
The Challenge
The commercialization of AI and proliferation of venture funding and hyper-growth pressures has put factors like time-to-market and engagement ahead of safety and efficacy. Diet and wellness devices and applications, for example, have become wildly popular in recent years. But there is little, if any, clinical evidence that they work. While they’re not necessarily causing harm, they’re essentially experiments in the wild.
Despite this, the healthcare and pharmaceutical industries are more mature than other verticals in terms of AI ethics. While there’s always snake oil on the market, from weight loss supplements to cancer cures and everything in between, mass production of medicine involves strict quality control, regulatory oversight and standards. But it took us about a century — counting from the emergence of industrial pharmaceuticals — to get there.
AI today is where pharma was in the middle of the nineteenth century; there are no established best practices, oversight, and few companies are doing it well. We have the tools and best practices to solve some of these problems, but we’re not enforcing them broadly.
Current Tools and Best Practices
Model governance, how a company tracks activity, access, and behavior of models in a given production environment is a key component for getting AI models safely into production and ensuring they stay safe and accurate over time. It’s important to monitor this to mitigate risk, troubleshoot and maintain compliance. This concept is well understood among data scientists and developers, but it’s also a thorn in their side, because current tooling is still in its early stages.
Rigorous testing and retesting is a good way to ensure models behave the same in production as they do in research. Looking at accuracy, bias and stability are factors that all practitioners should be analyzing on a consistent basis. Validating models before launching in new geographies or populations is the level of scrutiny that should be applied. Going beyond a single metric and applying behavioral testing and exploratory testing are other important best practices that should be applied today.
The same rules apply to keep models from degrading overtime, which is to be expected. Testing, automating retrain pipelines and measuring what was conducted before the model was deployed are all crucial to responsible and ethical AI. It’s far more likely to expect problems than optimal performance, and it’s the onus of businesses and practitioners to stay ahead of this.
Going Forward
Whether due to laws and regulations or high-profile missteps, we’re moving toward a more transparent future. One in which enterprises will be accountable when they say AI-powered services and products actually work. The good part is, we don’t need to wait for a data science equivalent of the Hippocratic Oath to start living and working by that ethos.
Even without an ethical framework or widely accepted solutions to AI bias, explainability or plain misuse, practitioners should acknowledge the limits of what they build. AI will be a major force for good in the 21st century, but it’s still in early days, and we must all put in the effort to do it right. As we’ve experienced, the alternative can be harsh and harmful.