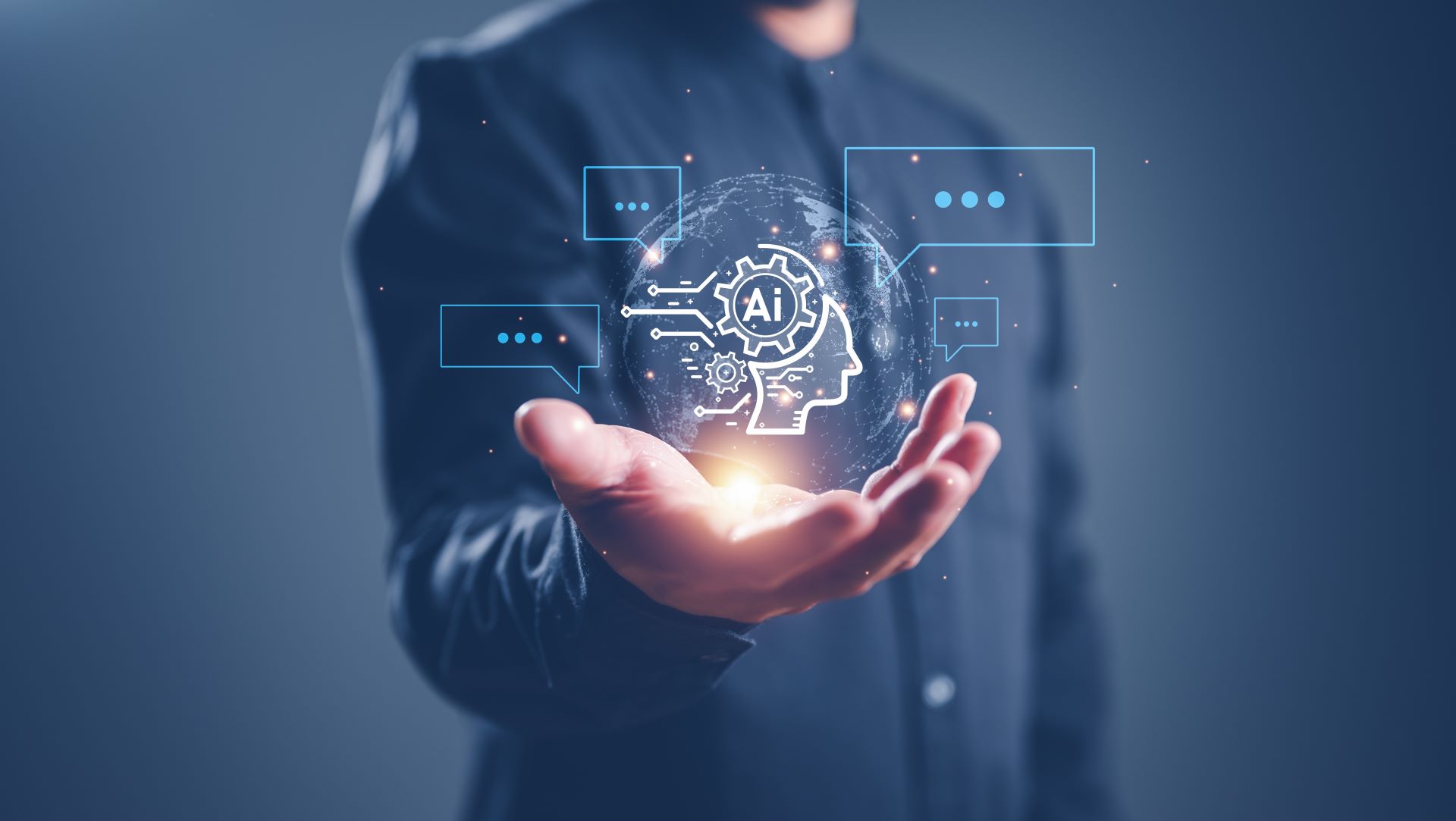
Unsurprisingly, data science and artificial intelligence (AI) have transformed enterprise decision-making. These technologies automate procedures that formerly relied solely on human intuition. Even though AI can analyze vast amounts of data and find patterns, human judgment is still vital.
The reason is that maintaining long-term corporate viability, ethical monitoring and strategy alignment depend heavily on human expertise. Although AI models are outstanding at optimizing for quantifiable results, they might ignore more significant economic factors, unforeseen consequences or ethical quandaries. By combining data-driven insights with human intuition, organizations can make more balanced judgments and avoid overreliance on technology.
The Balance Between Data and Human Intuition
Although predictive analytics highlights patterns in market dynamics, customer behavior and operational efficiency, a more comprehensive viewpoint that includes human intuition is necessary for final decision-making. It’s vital for enterprise leaders to evaluate AI-driven recommendations by considering long-term customer relationships, ethical issues and strategic goals.
Such cross-functional collaboration, which integrates many viewpoints from design, marketing, legal and product development, improves decision-making and ensures decisions align with corporate aims. Organizations that use predictive analytics to spark debate and improve strategy don’t rely on AI to dictate results.
Ethics is a critical example of the necessity for human intervention. AI models optimize for predetermined goals, which can clash with corporate accountability, regulatory compliance or customer trust. By incorporating human input into decision-making, organizations can spot problems before they become severe enough to give rise to regulatory attention or harm their reputation.
Dangers of Overgeneralizing Data Science Trends
A primary danger of AI-driven decision-making is the propensity to extrapolate patterns from past data. Although machine learning models are competent at identifying patterns, they naturally lack context awareness. Relying too much on AI recommendations may result in choices that do not consider shifting regulations, consumer behavior, or external market conditions.
A notable instance of this risk was legal action by the Federal Trade Commission (FTC) against Adobe for alleged deceptive practices in its web checkout flow. Adobe is known to run A/B tests to continually improve its products and services. Iteratively A/B testing and optimizing for short-term revenue metrics could reshape a web experience to “reduce friction,” ultimately limiting the visibility of early termination fees. This practice risks customer trust and brand reputation.
Additionally, localized trends don’t necessarily transfer to larger markets. For instance, a recommendation engine can determine that a particular product category is regularly bought by high-value clients and modify marketing strategies accordingly. Giving these suggestions too much weight could turn off other target audiences with differing tastes, lowering engagement and short-term brand loyalty.
Using Human Knowledge in Decision-Making Procedures
Structured frameworks that balance AI insights and human skills help reduce the risks of making decisions solely based on data. Companies can avoid optimizing for incorrect evaluation criteria at the expense of long-term sustainability by establishing a disciplined decision-making process that considers ethical considerations and input from various stakeholders.
Uber’s previous pricing strategy is a well-documented example of algorithmic bias being corrected by human intervention. In its early incarnations, Uber’s surge pricing strategy depended solely on algorithmic changes based on demand. This practice caused sharp price increases at times of high demand and emergencies, infuriating customers. The algorithm overlooked long-term brand reputation and ethical issues while optimizing for short-term income. A change in leadership in 2017 restored human judgment to the decision-making process, resulting in modifications that put equity first while preserving the company’s viability.
Promoting discussion among data scientists, subject matter experts and corporate leadership fosters a culture of accountability. Organizations can benefit by viewing AI and data science as analytical partners contributing insights to human-led discussions rather than absolute decision-makers. Businesses can also promote transparency by entertaining opposing views, eventually reviewing their choices, and improving their tactics.
The Role of Ethical Considerations and Qualitative Measures
Research from the National Institutes of Health emphasizes the importance of structured human-AI collaboration in decision-making. It also warns against overreliance on AI by highlighting the need for frameworks that integrate human judgment to mitigate biases and enhance reasoning.
Companies that only use quantitative performance metrics run the danger of maximizing profits in the near term at the expense of long-term strategic goals. Unintended repercussions could occur if qualitative factors are not included with numerical key performance indicators (KPIs).
Social media sites that focus solely on engagement, for example, may unintentionally spread sensational or polarizing material, harming user welfare and business reputation. Similarly, by giving some products too much priority, an e-commerce platform primarily concerned with boosting revenue per transaction may inadvertently drive away loyal customers.
Organizations can incorporate qualitative indicators and quantitative data to preserve ethical and strategic alignment when defining success. Metrics include revenue growth, customer satisfaction, brand perception and regulatory compliance. Ethical oversight is also required to prevent manipulative business activities that might harm a company’s brand or have legal ramifications.
Future Trends and Best Practices for AI-Driven Decision-Making
More cooperation between AI systems and human decision-makers, as opposed to complete automation, is likely where the future of data science lies. According to McKinsey, AI is reshaping workforce dynamics, requiring companies to upskill employees and integrate human expertise. Organizations will increasingly use structured decision-making frameworks that integrate human judgment as they realize the limitations of solely data-driven strategies. Here are four major trends influencing the development of AI-driven decision-making:
- Increased regulatory oversight. Governments and regulatory agencies are expected to enact more stringent rules regarding algorithmic transparency, data privacy, and AI ethics. Early compliance with these rules will give businesses a competitive edge.
- Integration of qualitative and quantitative metrics. Companies will place a high value on a fair performance review process considering long-term brand impact, customer experience, and financial results.
- Cross-functional cooperation in AI development. Data scientists will increasingly collaborate with product managers, designers, and legal specialists to ensure that AI-generated insights complement overall business goals.
- Ongoing assessment of AI-driven systems. Organizations will regularly evaluate and improve AI models to avoid bias, guarantee relevance, and uphold ethical norms.
The Future of AI-Driven Decision-Making
Even though AI-driven insights improve decision-making by spotting patterns and increasing productivity, human judgment is still crucial. Data science models offer valuable inputs but they cannot holistically account for strategic vision, ethical issues or unintended repercussions.
By incorporating human expertise into the decision-making process, organizations can ensure AI-driven initiatives align with long-term business goals, consumer expectations and corporate values. When companies make responsible, well-informed decisions, they more likely adopt a systematic strategy that promotes discussion, ethical monitoring, and a combination of quantitative and qualitative performance evaluations.
Organizations that value cooperation among data scientists, business executives and subject matter experts will be best positioned to use data science for long-term growth as AI develops. Ultimately, the future of corporate decision-making involves integrating AI with human judgment to promote ethical accountability and tactical flexibility instead of favoring one over the other.